PEOPLE PLUS MACHINES
The role of Artificial Intelligence in Publishing
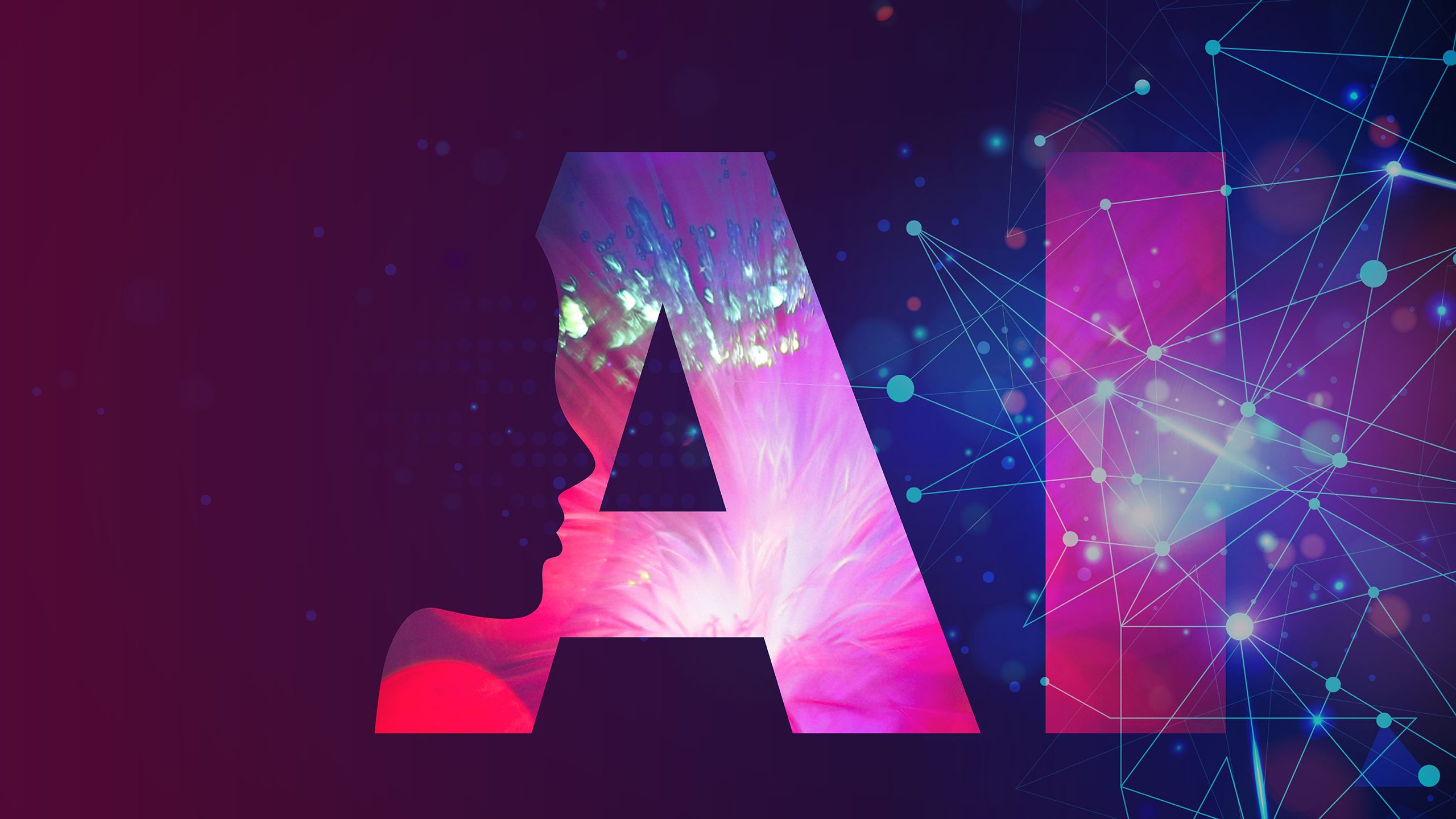
Executive Summary
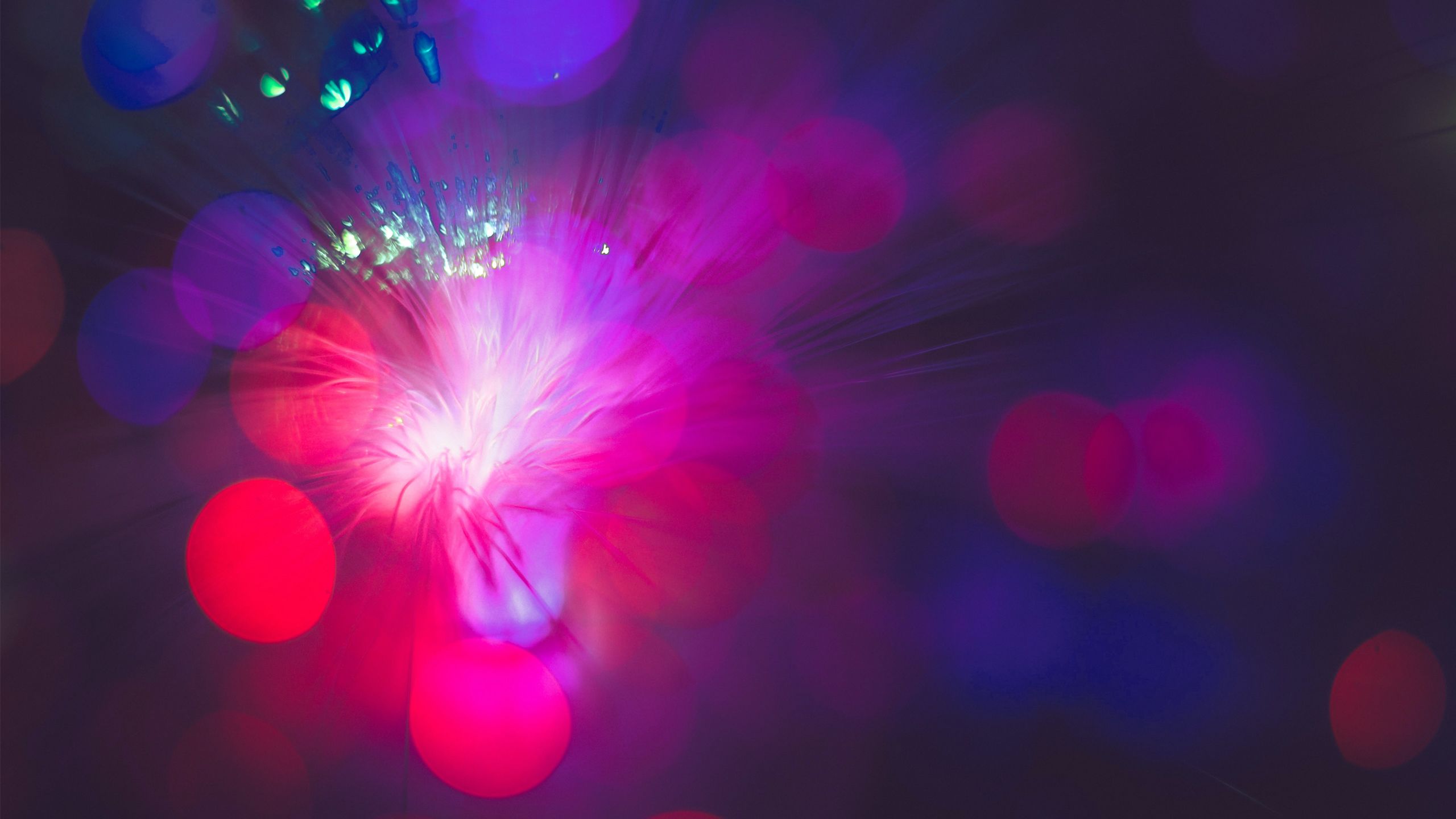
The UK government’s Industrial Strategy places heavy emphasis and public investment on Artificial Intelligence (AI) as a driver of business innovation and future productivity growth. The Publishers Association asked Frontier Economics to provide an evidence-based assessment of the role of AI in the publishing sector.
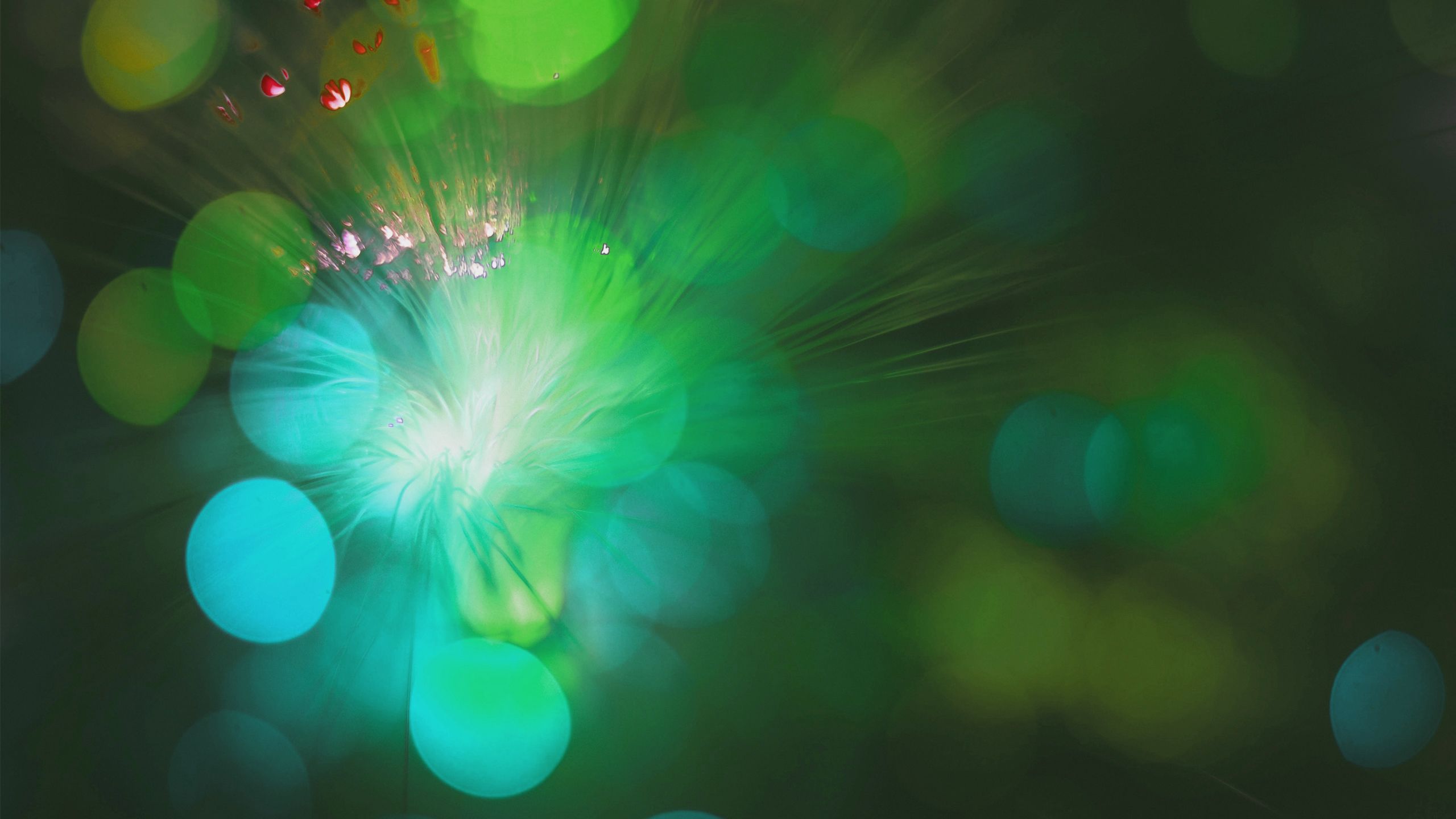
To the best of our knowledge, this report is the first systematic analysis of AI in publishing for the UK. Based on sector interviews, case studies and an industry-wide survey, we develop a use case taxonomy of AI for publishing, and evidence on publishers’ behaviours and attitudes around AI investment.
Key insights and policy recommendations
- AI is being applied throughout the value chain by some academic, education and consumer publishers to drive benefits for their organisation (such as improved IP protection, content discoverability, market prediction and other strategic insights) and for their customers (by conducting routine search and summarisation tasks and generating new insights, AI is freeing up researchers, authors, teachers and consumers to focus on value-add or creative tasks).
- Overall, the majority of publishers, irrespective of size and sector, consider that AI will be important over the next five years. Of the publishers we surveyed that are already investing in AI, the majority have realised benefits, and all expected to do so within the next few years.
- AI investment in the sector has just begun. Larger publishers are leading the drive. Most investment began within the last three years. Publishers use small internal AI research teams, and collaborations with AI-tech start-ups and university researchers.
- To increase publishers’ AI investment levels and drive increased consumer benefits, the sector must overcome a number of investment barriers, including a lack of technical AI skills and general awareness of the benefits of AI, difficulties implementing AI solutions with existing IT infrastructures and across siloed work streams, the importance of legal certainty regarding UK Intellectual Property (IP) Law, and for smaller publishers, the significant upfront investment costs associated with AI research and implementation.
- We recommend that the industry and government in the UK work together to raise awareness of key AI investment issues, promote engagement and identify policy and other solutions to address them. Key policy areas include: ensuring legal certainty regarding UK IP law; promoting collaboration between publishers, AI-focused SMEs and academia; and helping SME publishers access AI finance and skills.
The remainder of this summary pulls together key insights from extensive desk research and primary evidence gathered through conversations with publishers, an industry survey and case studies on:
- Defining AI (and a key aspect of AI, Machine Learning (ML)) in the context of the publishing sector;
- Developing a ‘use case’ taxonomy of AI in publishing linking applications of AI to user and wider benefits;
- Identifying the barriers to AI investment and adoption faced by publishers;
- Publishing and AI in the future, particularly around how the use of and benefits from AI in publishing are likely to evolve; and
- Recommendations for how policy can best support publishing in overcoming investment barriers and driving benefits.
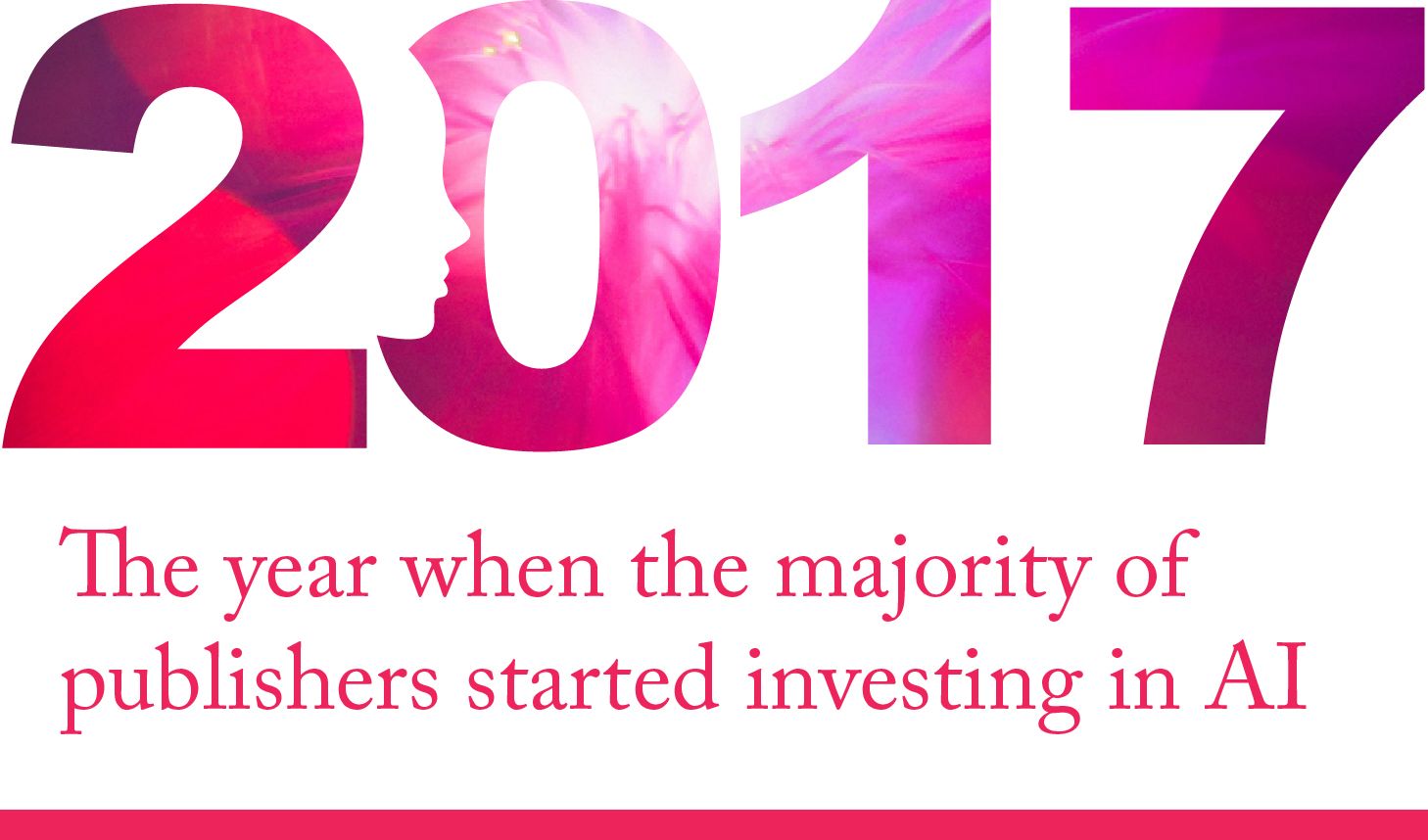
Defining publishing and AI
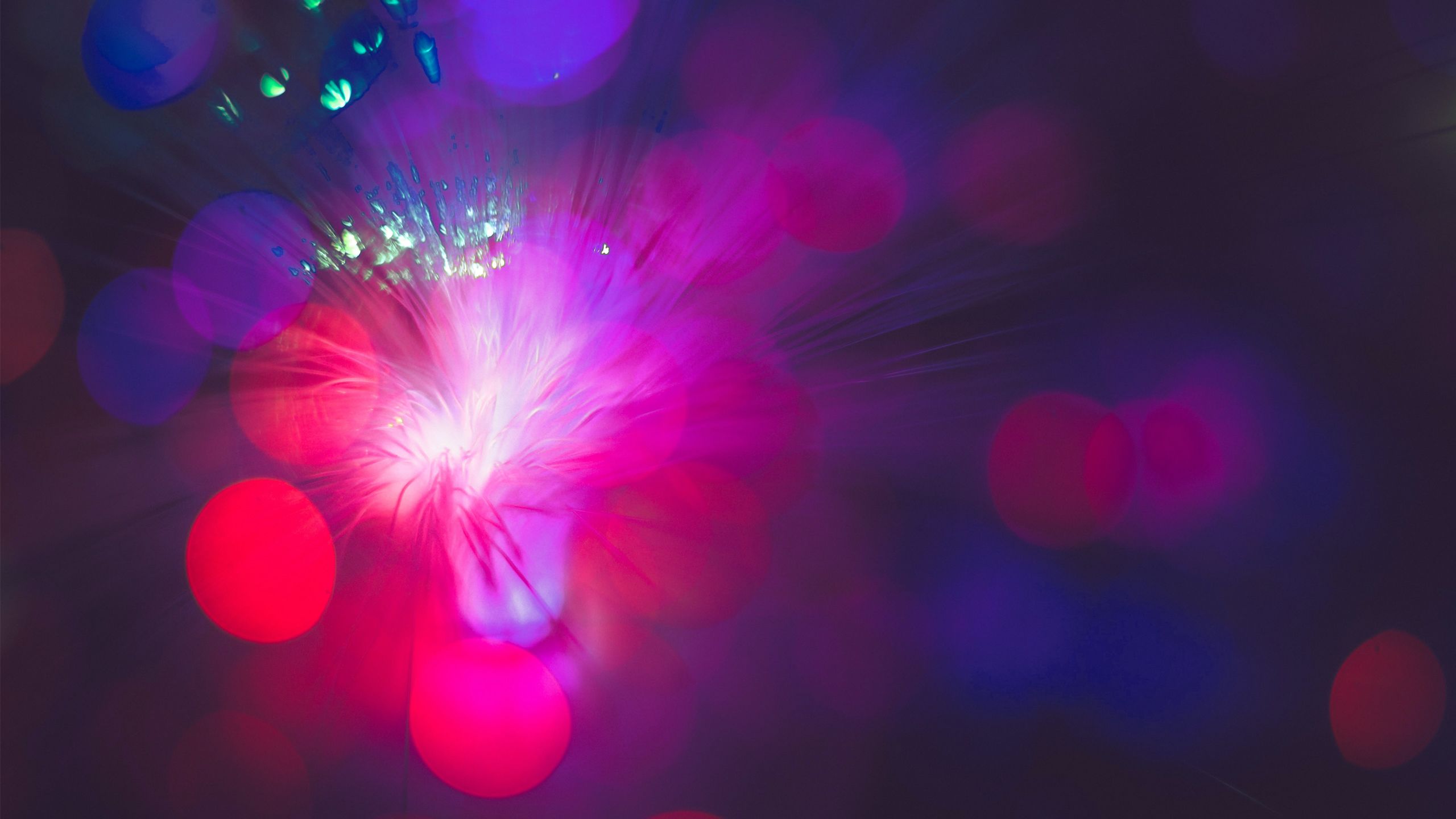
The scope of this report includes academic, education and consumer publishing. Our analysis does not cover newspaper, catalogue and magazine publishing, or software and computer games.
A key finding is that there is no single definition of AI: the term AI is used broadly to cover a range of applications. Our working definition for this study was developed in consultation with publishers, recognising that the scope of AI is constantly changing and can be interpreted differently depending on the specific application.
Defining artificial intelligence for the publishing sector
Artificial Intelligence is a broad term that covers multiple technologies which enable computers to sense, comprehend, act and learn. All current uses of AI in the publishing industry involve Machine or Deep Learning - either alone, or in combination with other technologies such as Natural Language Processing (NLP), Voice Recognition or Computer Vision.
Our approach and evidence base
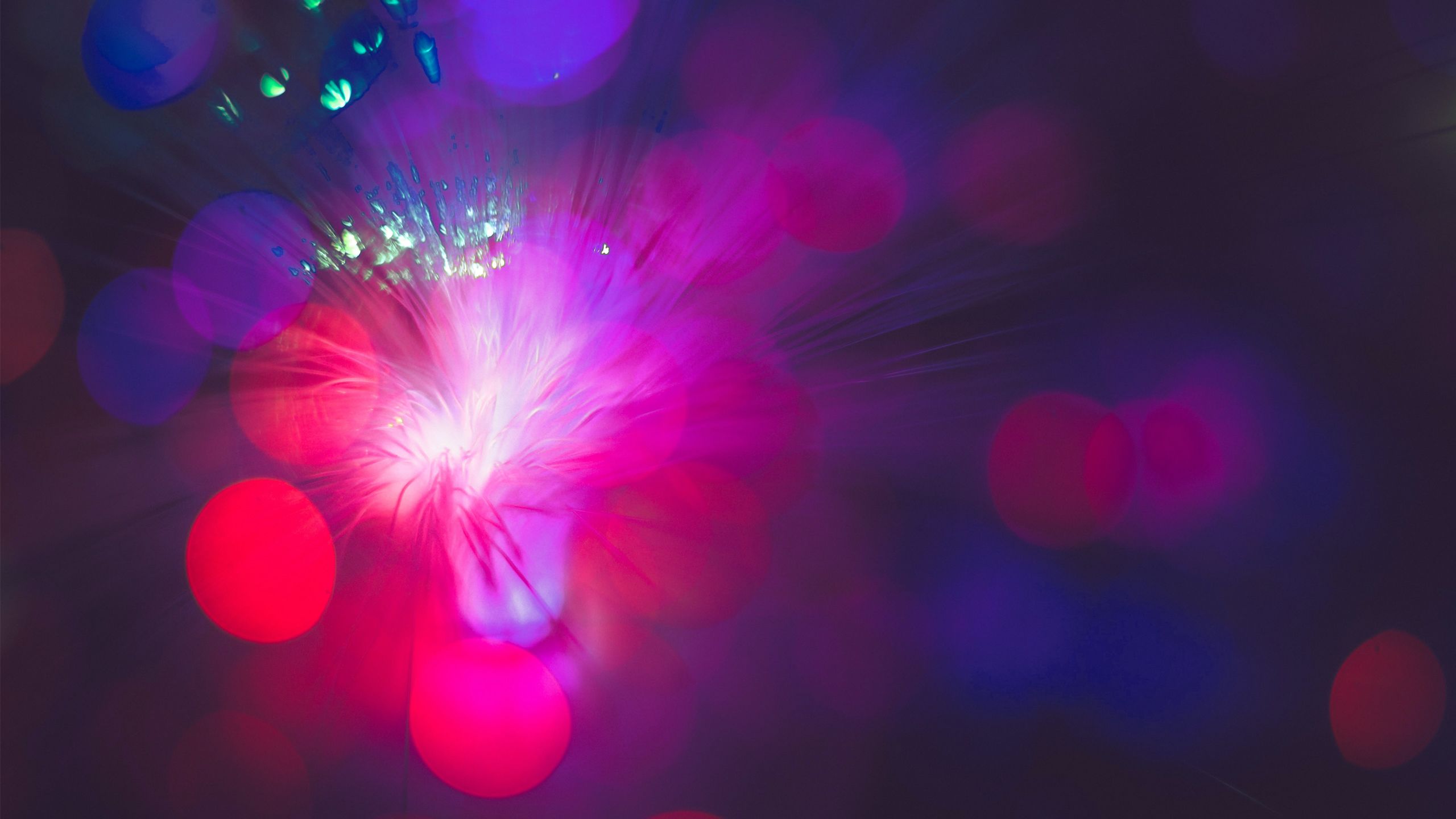
The evidence and analysis for this study was generated in four steps (see below) and relied on extensive primary and secondary evidence gathered over the period November 2019 to March 2020.
The primary evidence was gathered through:
- a series of seven telephone interviews with stakeholder publishers;
- an online survey sent to all 135 members of the Publishers Association which generated 31 responses, including around two-thirds of the Association’s larger members; and
- three in-depth case studies constructed through telephone interviews and desk research.
The secondary evidence was compiled through an in-depth literature review covering AI use cases and the relevant regulatory issues concerning AI investment for the publishing industry.
An AI taxonomy for publishing
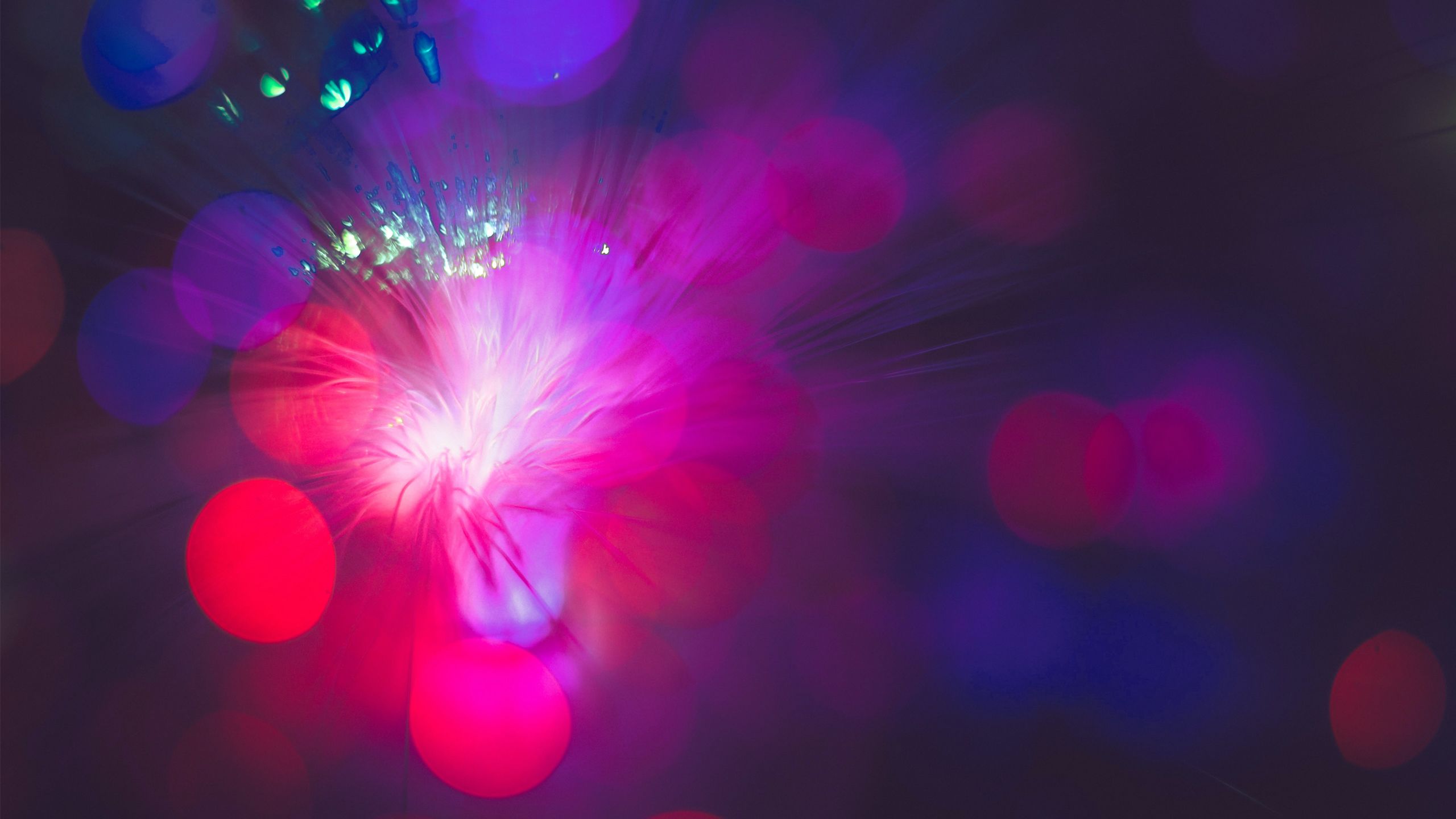
Our taxonomy is structured around ‘value chain – technology – benefits’. This reflects how most publishers think about AI investment decisions in their organisation.
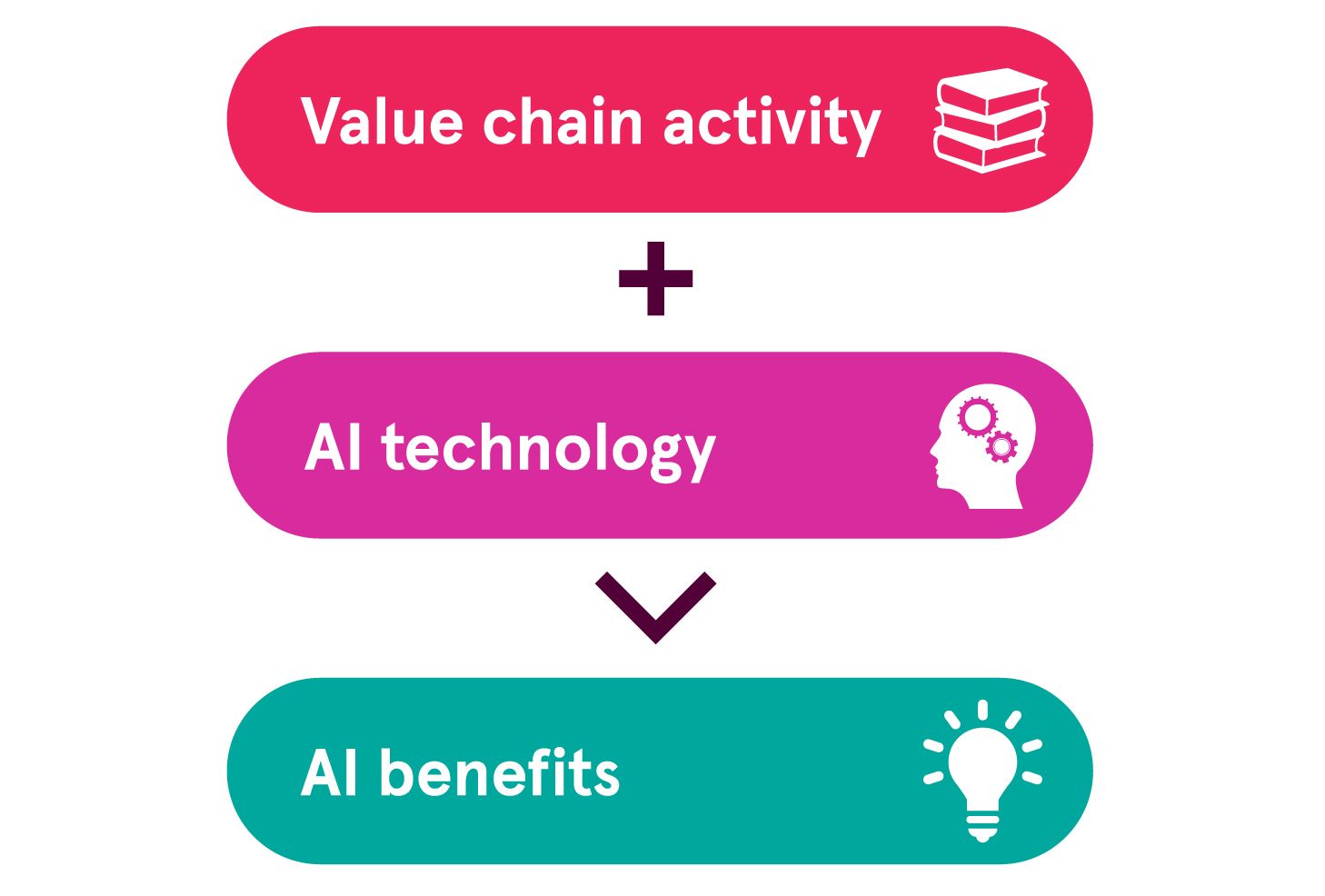
We learnt that most investment decisions are benefit driven – ‘Can we address this issue, be more effective with AI, deliver significant benefits to our customers?’. In some organisations, technology-fit is also important – ‘Can we apply AI to drive benefits within the current organisational structure / with these resources?’.
The role of AI in publishing can largely be represented by the summary taxonomy below. The main report provides more detail including nuances of the taxonomy for the specific academic, consumer and educational publishing sub-sectors.
The taxonomy highlights that the AI technology focus for publishing is a combination of text and image recognition technologies with machine and deep learning. AI can be applied in a wide range of ways throughout the publishing supply chain to generate benefits both to publishers and consumers – for example, time-saving benefits, speeding up, improving the quality of and providing additional insights for innovation activities, improving operational efficiency and cost savings, and improving the customer experience.
These initial publishing benefits should generate subsequent wider benefits for the economy as a whole, including companies’ improved ability to compete through innovation and efficiency gains, increased academic attainment levels, lower cost and faster medical break-throughs generating patient well-being benefits, and the well-being benefits associated with improved customer satisfaction.
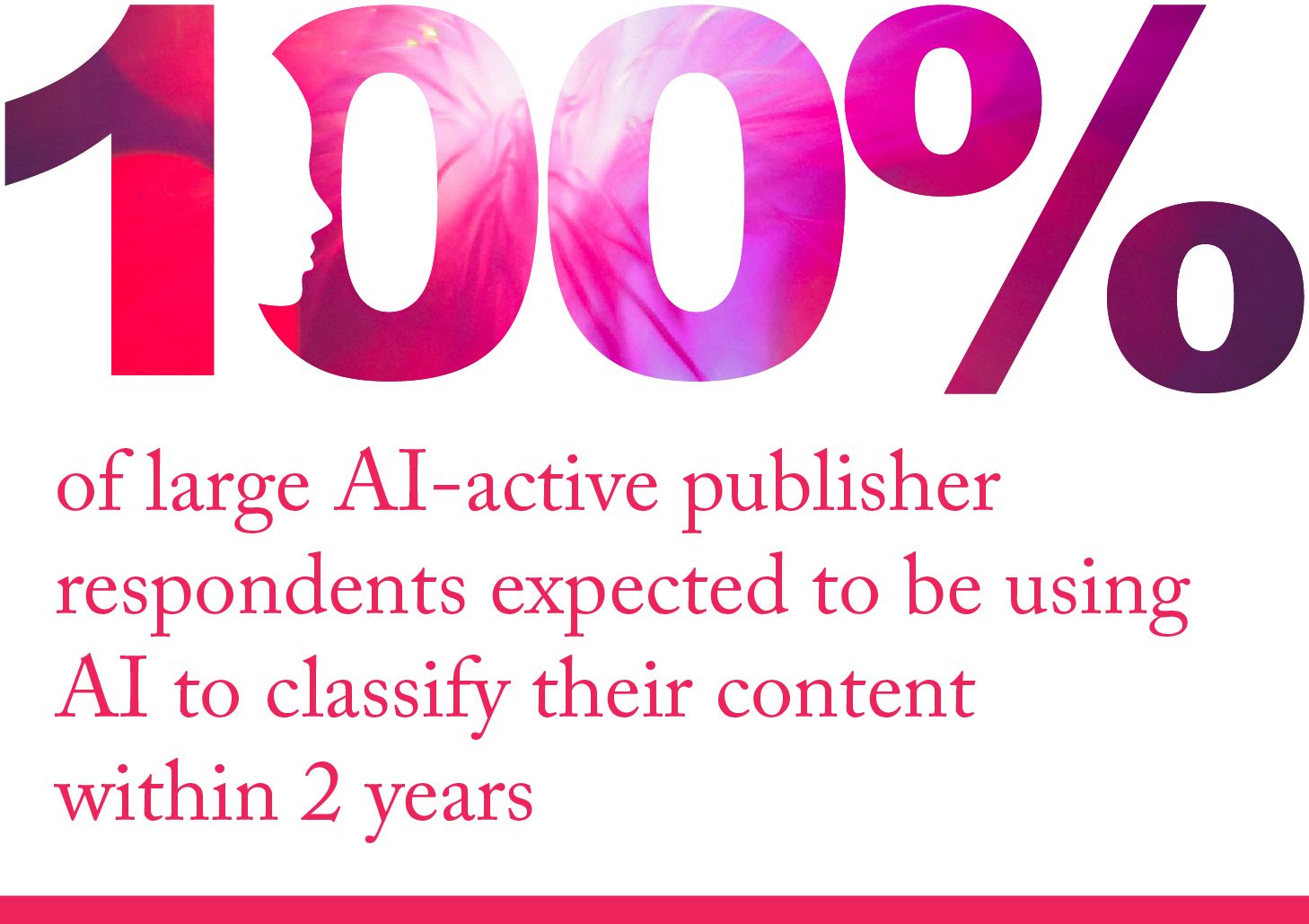
Evidence on AI investment activity
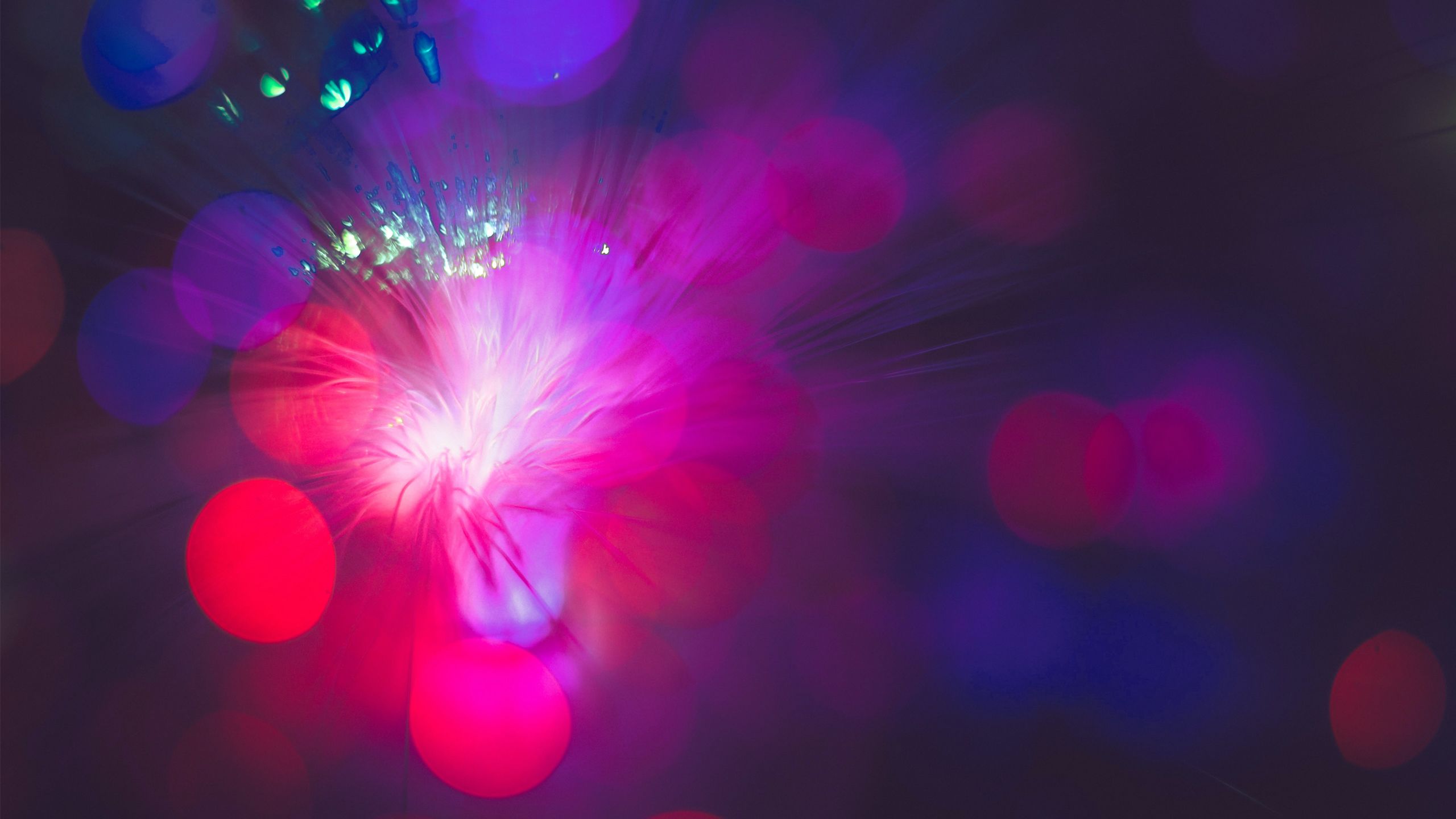
The online survey provides a current snapshot on the nature of AI investment by publishers. The responses we received from large publishers (employing at least 250 FTE staff worldwide) appear to be representative of larger UK publishers. We received only one response from a smaller publisher that was already investing in AI. Therefore our survey insights focus on larger, ‘AI-active’ publishers. More detailed research is needed for smaller publishers to fully understand their AI-related behaviours.
Our findings suggest that though a few publishers were investing in AI as far back as 2014, most of those currently investing first did so in 2017. Total investment in AI is increasing year-on-year. The majority of publishers currently investing in AI have small internal teams of around 1 to 5 staff focused on AI research, with around half of these based in the UK.
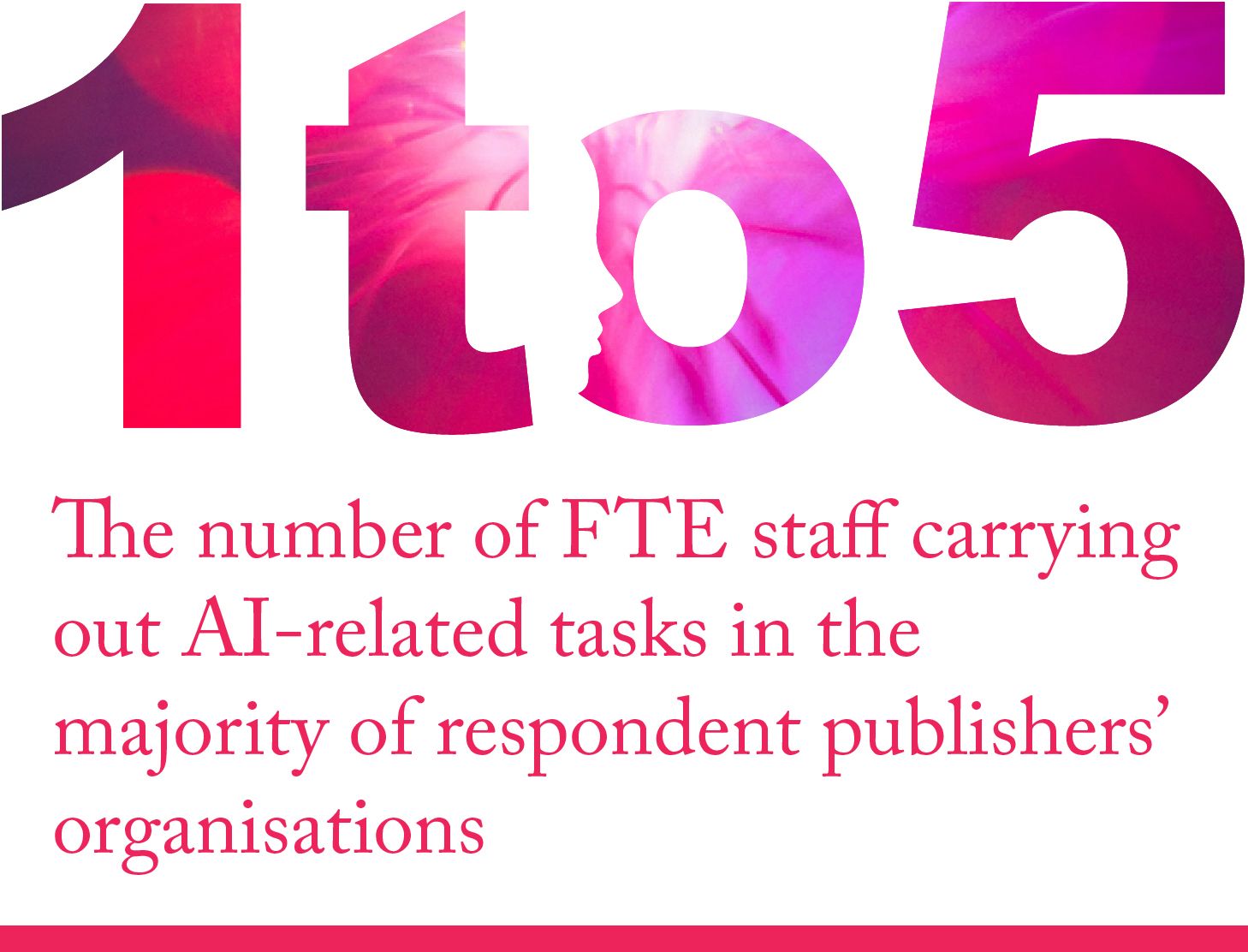
Almost all of the large publishers who responded said they were currently using AI in their organisation, or were exploring how it could be used. In terms of our taxonomy, the responses reveal that:
- AI is being applied throughout the publishing value chain, with perhaps less emphasis to date on customer service. Large publishers are most commonly using AI to acquire and develop new content (45% of large AI-active publishers). In the near future this may change to AI being most commonly applied to provide marketing and sales solutions: three-quarters of large publishers are already using or plan to introduce marketing and sales solutions within the next two years.
- The most common application of AI in publishing at present is content classification (for example, using meta data tagging to improve the discoverability of their content in conjunction with recommendation engines). Other common applications include using AI to identify market trends and support recommendation platforms.
- Two-thirds of these large AI-active publishers have already realised benefits from their AI investments. Within two years, all large AI-active publishers expected to have realised AI benefits. The most commonly cited realised benefits include improved IP protection and risk management (for example from an improved ability to detect plagiarism), increased competitive advantage and improved strategic insight.
The case studies provided further evidence of how AI can improve the discoverability of relevant academic content through metadata tagging and the development of research engines, helping support higher quality research outputs. We also show how AI can develop educational content to deliver improved insights to students and time saving benefits to teachers, enabling them to spend more time with their students.
One case study also illustrated how AI has been used to generate new publishing content, applying ML algorithms to the vast catalogue of scientific research. This application could unleash significant benefits in the field of academic research, helping rapidly select, categorise and summarise significant quantities of text data both more quickly and, potentially, more accurately than can be done by human researchers. We also found some examples of AI being used to generate consumer content. While stakeholders consider it unlikely that publishers will be marketing AI-generated fiction novels in the near future, there is considerable innovation activity in this area. More generally, these examples of AI generated content in publishing illustrate the potential for AI to increase writers’ ability to create, through to freeing them up from routine research tasks and/or rapidly providing them with new scientific or creative insights.
Case study 1
AI and academic content generation (Springer Nature)
Springer Nature published ‘Lithium-Ion Batteries: A machine-generated summary of current research’ in 2019. The book, which took 1.5 years to develop and produce, represents one of the publishers’ most significant AI- projects to date. It is an example of publishers collaborating with AI and data experts to bring in the required AI expertise: the project brought together the expertise of Springer Nature’s Data Development team and their global area experts (chemistry and materials science), with the University of Frankfurt, and Digital Science (a science service partner).
The project is also an example of AI investment being benefit driven. The key motivation for producing the book was based on Springer Nature’s own research which showed that ‘information overload’ is a significant problem for researchers: for example there is no available subject overview that a person can run through in a couple of days to understand a problem. Another insight that motivated the project was their understanding that modern problems - such as meeting the UN’s Sustainable Development Goals require inter-disciplinary working, rather than siloed working practices. AI has the ability to cut through disciplines.
As well as these ‘user benefits’, the project intends to generate industry spill-over benefits. All of the code used to create the book has been placed in open-source enabling researchers to continue to develop the technology. Springer Nature hopes that this will lead to a greater level of information sharing to innovate more efficiently and to create a standard that will ultimately be accepted by various communities and markets.
The book itself was generated by applying AI technologies (a combination of machine learning and NLP technologies) to an initial long list of 1,086 publications identified through keyword searches. A simplified overview of the book generation process and key technologies employed is as follows:
- Document clustering and ordering: First, clustering technology was used to identify the specific contribution and scope of each input document, with a selection process used to prioritise and order documents by relevance.
- Extractive summarisation: Next, auto-summarisation technologies were employed which create excerpts from the documents and form the basis of the book’s subsections.
- Paraphrasing and the generated extracts: Finally, aggregation and paraphrasing techniques were used to improve the readability of the content.
The approach adopted by Springer Nature is considered conservative in order to preserve the audit trail. At any time, the reader is able to link through to the underlying source (something that was monitored by Springer Nature closely). ‘Black box’ AI solutions need to be considered and applied carefully by publishers, especially in the areas of peer review where audit trails are fundamental to judging quality. Similarly for scientific research, researchers require accurate reporting with a full audit trail. Springer Nature hopes that its first output in this area initiates a critical starting point for continuing research.
Source: Frontier stakeholder interviews and Beta Writer, 2019. Lithium-Ion Batteries: A machine-generated summary of current research.
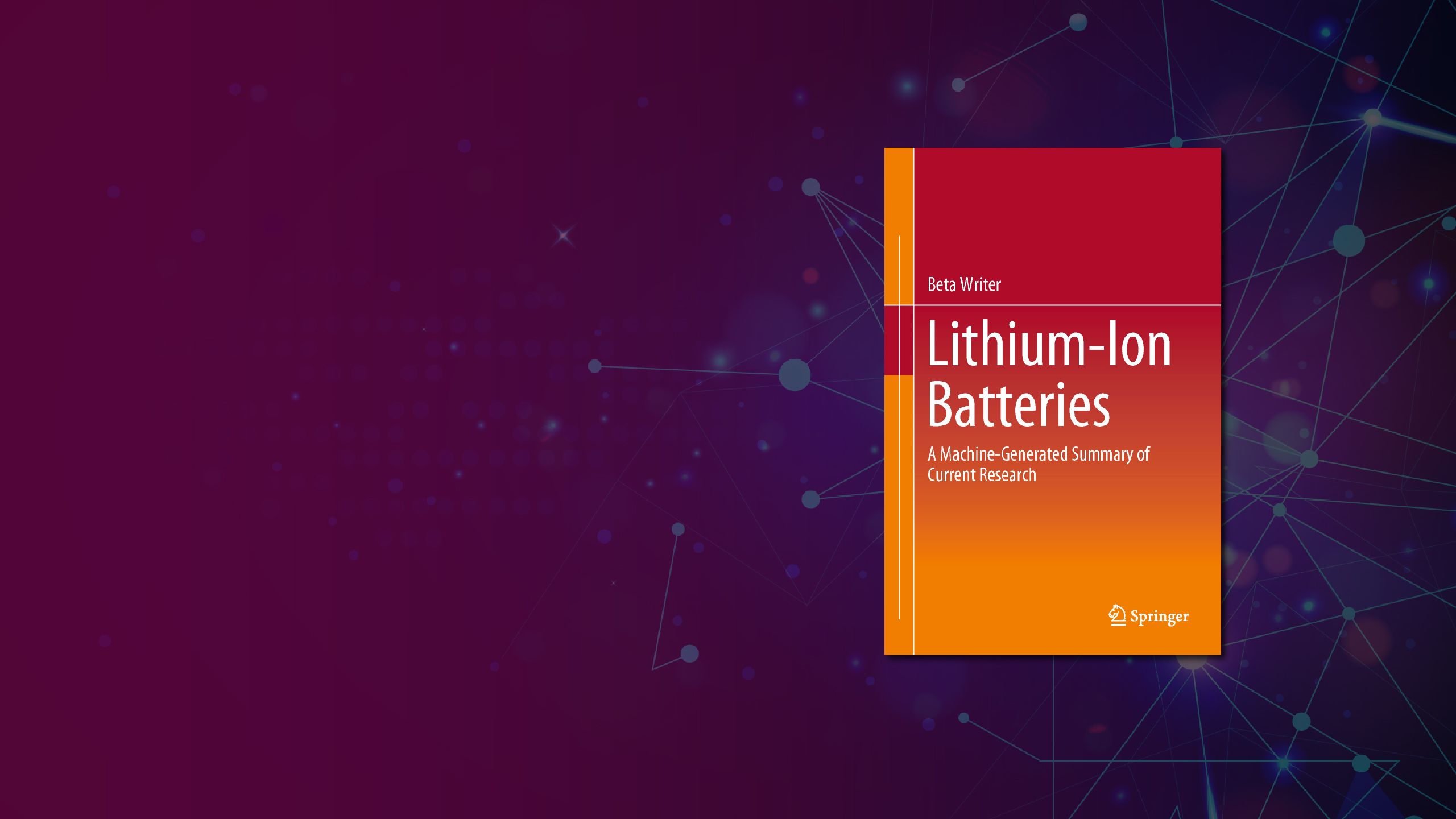
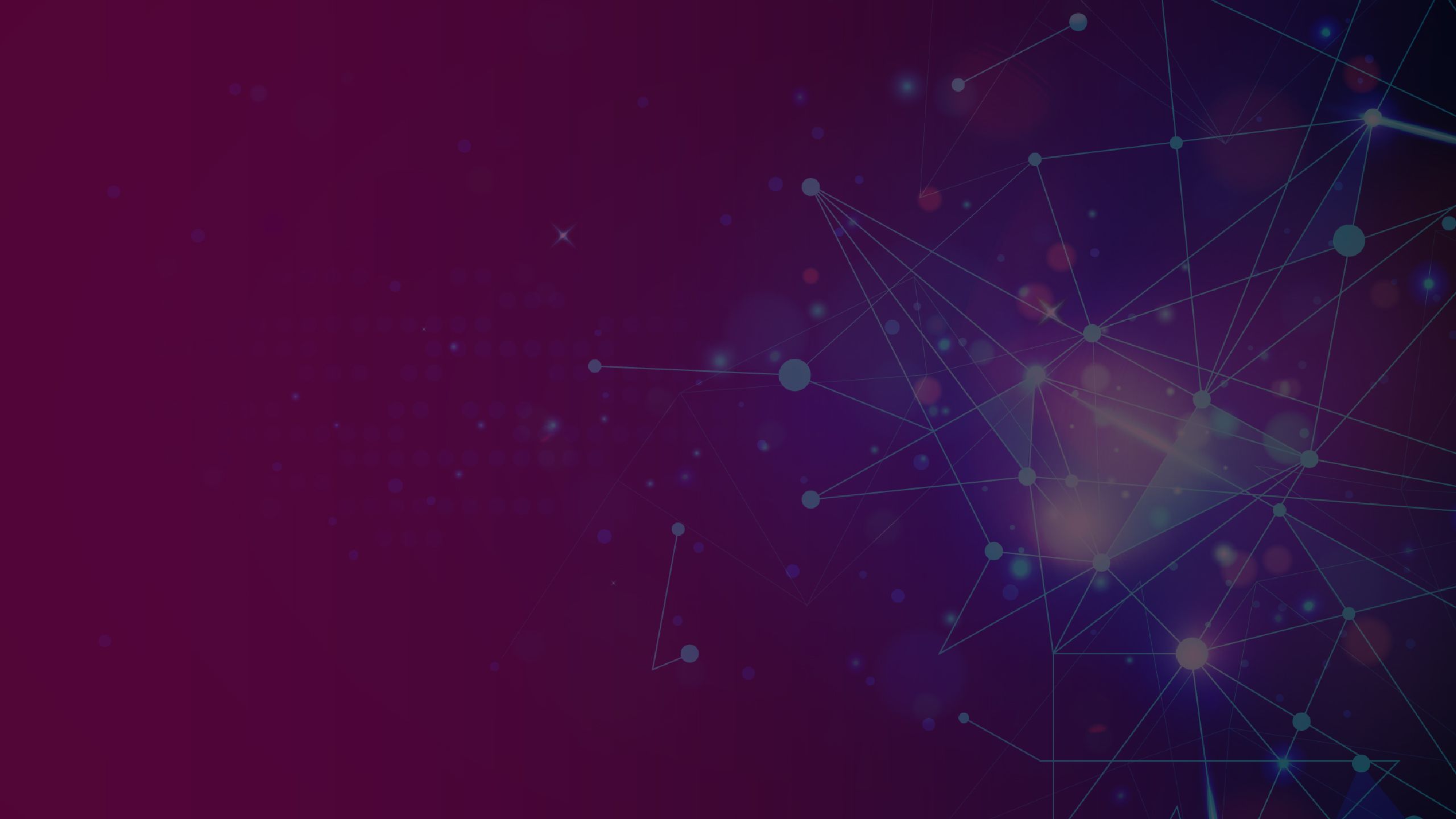
AI investment barriers
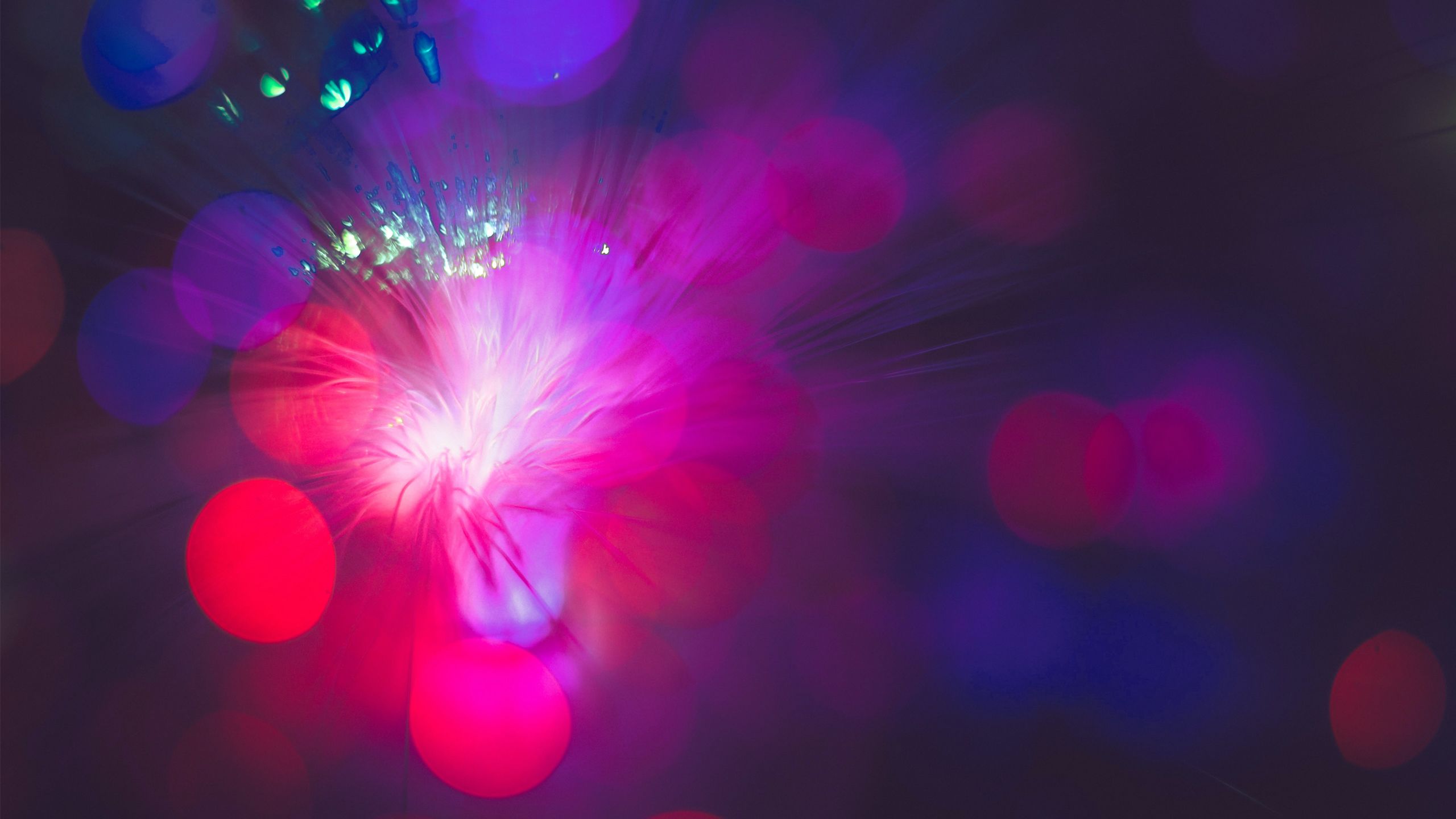
The survey evidence suggests that a lack of AI-related skills and difficulties applying AI solutions with existing IT infrastructure are the most common AI investment barriers faced by large AI-active publishers.
We found evidence of publishers overcoming skills and technology barriers through collaboration with external research organisations: half of the large publishers we surveyed on this issue consider themselves largely dependent on external expertise for acquiring AI skills and technology.
Lack of awareness of the potential benefits of AI also appears to be a significant barrier for large AI-active publishers. We understand this may be linked to a lack of scientific knowledge by investment decision-makers, and may also be attributable to the fast rate of technological change currently seen for AI. The evidence we gathered suggests that overcoming this awareness barrier could also unlock several other issues identified by some stakeholders, such as organisational barriers (e.g. willingness to invest in AI solutions that cut across organisational silos, requiring buy-in from multiple decision makers).
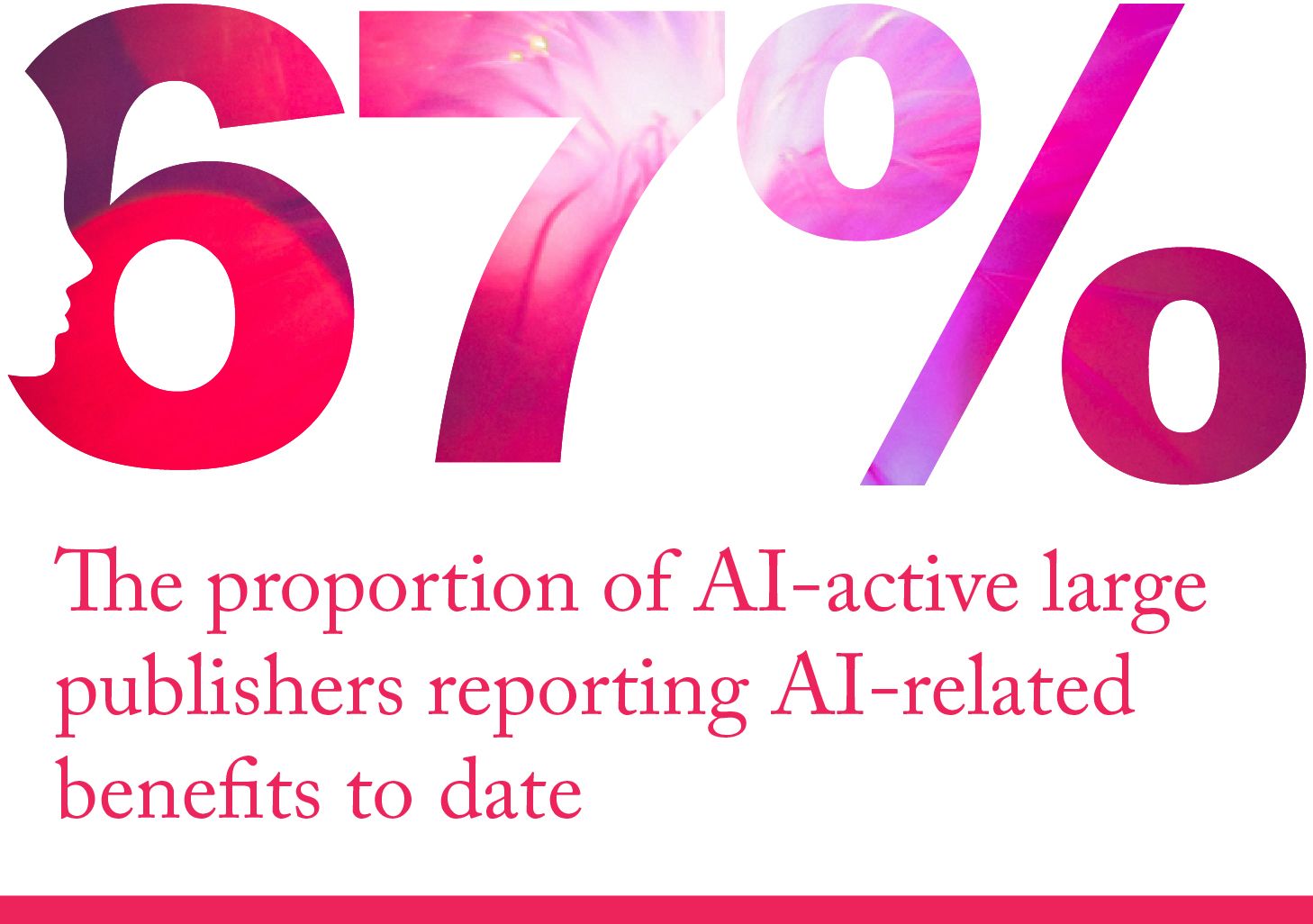
Though the direct evidence we have on barriers facing SME publishers is more limited, we did find that large upfront costs associated with researching and implementing AI solutions may be prohibitive for smaller publishers.
Case study 2
AI and publisher content recommendation (Elsevier and Taylor & Francis)
Elsevier’s Mendeley Suggest and Taylor & Francis’s partnership with UNSILO illustrate the value of using AI to help academic researchers identify relevant research that will feed into future published research outputs.
The focus of Taylor & Francis’s three-year partnership with UNSILO (a Denmark-based technology solutions company - specialising in providing publishing solutions - and part of Cactus Communications) is around generating meta-data for Taylor & Francis’s content. This has enabled Taylor & Francis to create a recommendation engine using UNSILO’s Recommend product. This recommendation engine is provided to academic library customers to help their users identify relevant Taylor & Francis content. It is also improving the quality of the meta-data it generates for content that Taylor & Francis supplies to indexing companies such as Google Scholar. In this case, AI is used to read academic content (using NLP) and subsequently creates a knowledge base to identify the most useful keywords that can be used as tags. There is a feedback loop enabling the algorithm to be fine-tuned.
Although Taylor & Francis does not formally track the direct benefits of its AI investment in these areas, it expects that this higher quality data is enabling researchers identify more relevant content in a shorter time period, and Taylor & Francis to increase sales volumes by improving discoverability.
Elsevier acquired Mendeley (a free reference manager and academic social network) in 2013. The Mendeley Suggest feature within the platform uses ML to generate recommendations for users on what to read and who to collaborate with. ML analyses data on the interests of similar users (explicitly stated or inferred from papers they are reading). A process of Collaborative Filtering then enables Mendeley to predict user preferences for a set of items based on past experience. The diagram below is a simplification of the most commonly used collaborative filtering algorithm, as an example of the process.
Mendeley’s own use case studies provide evidence of a range of user benefits, including helping researchers from ETH-Zurich and ETH-Bibliothek to collaborate across ‘boundaries’,[1] helping the Institut Pasteur discover new research,[2] and helping the International Food Policy Research Institute to track and promote its authors’ papers.[3]
[3]https://www.elsevier.com/__data/assets/pdf_file/0004/69673/IFPRI_case_study_v5.pdf
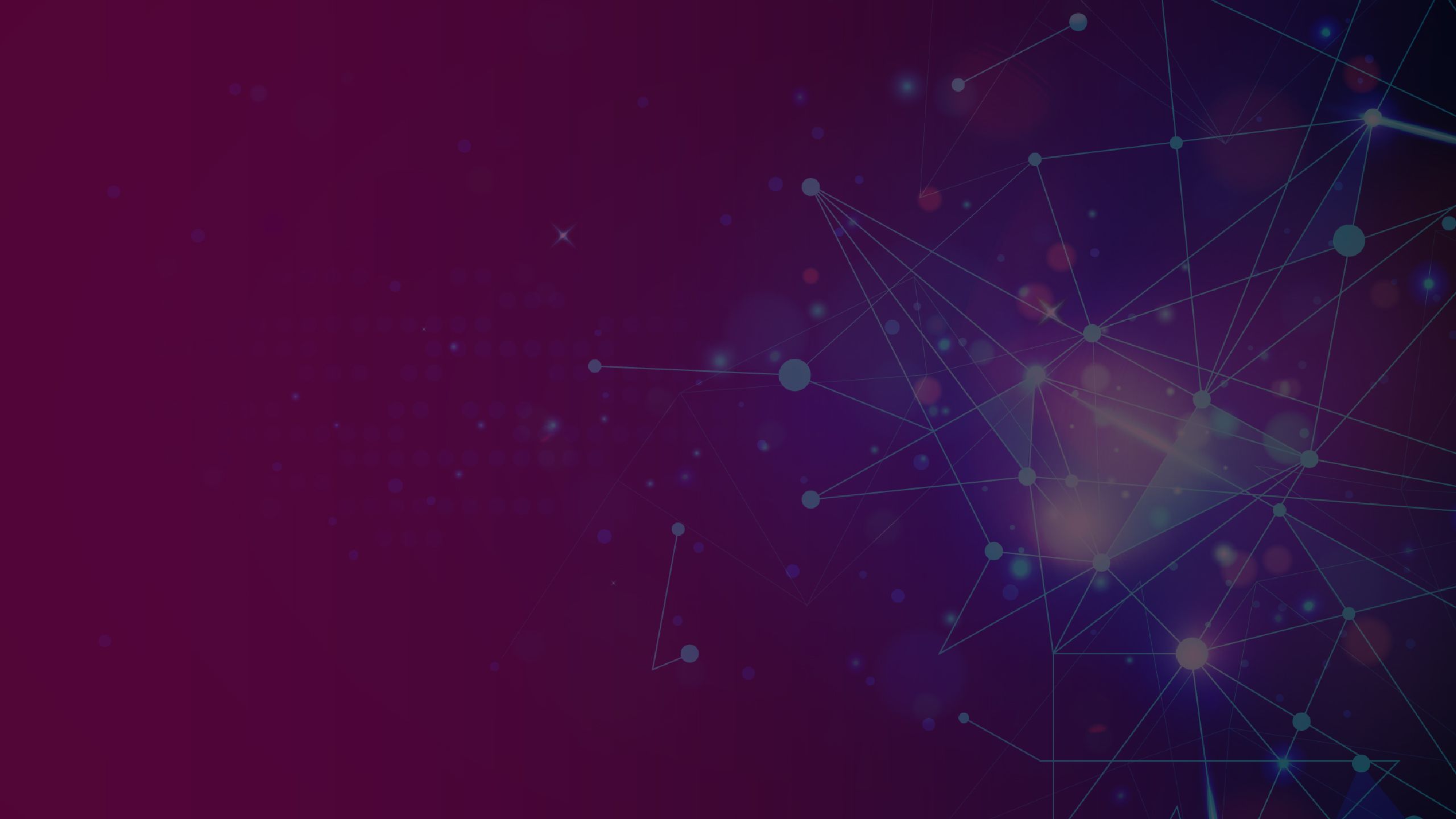
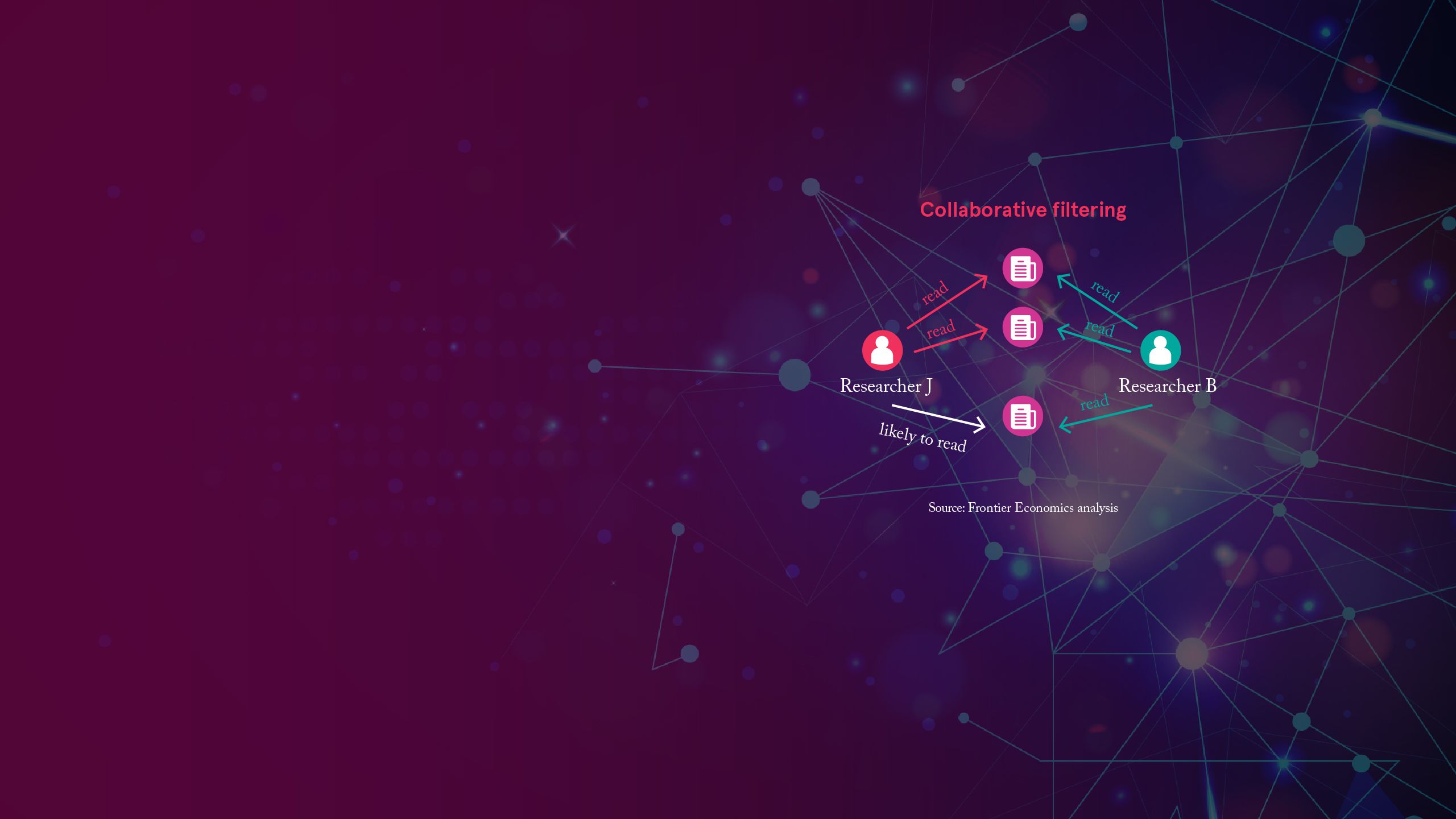
Publishing and AI in the future
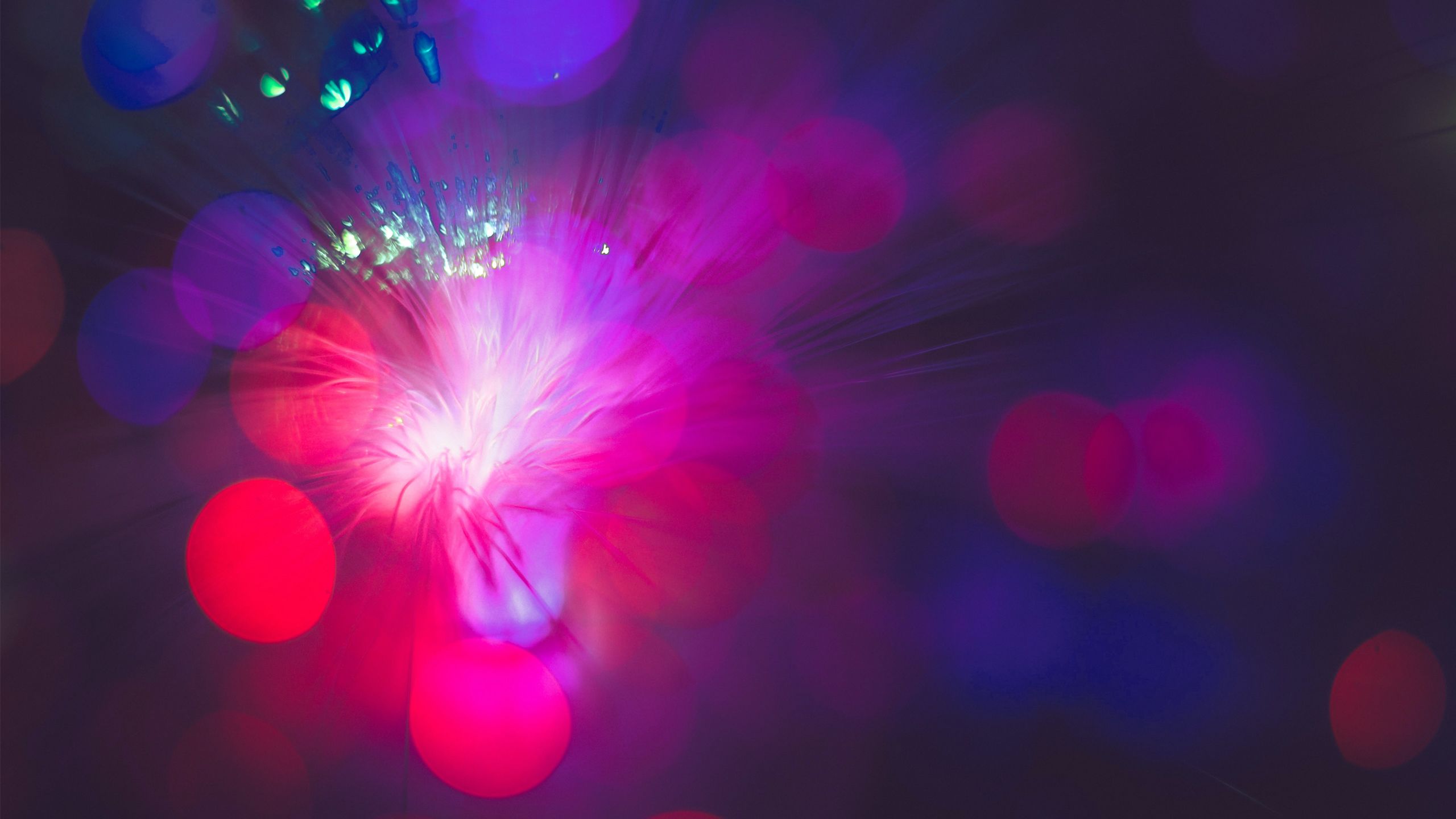
We asked publishers about how important they felt AI would be for the industry in the next five years. On a scale between 0 and 100, publishers on average scored the future importance of AI for the sector at 69. This suggests a high degree of future importance.
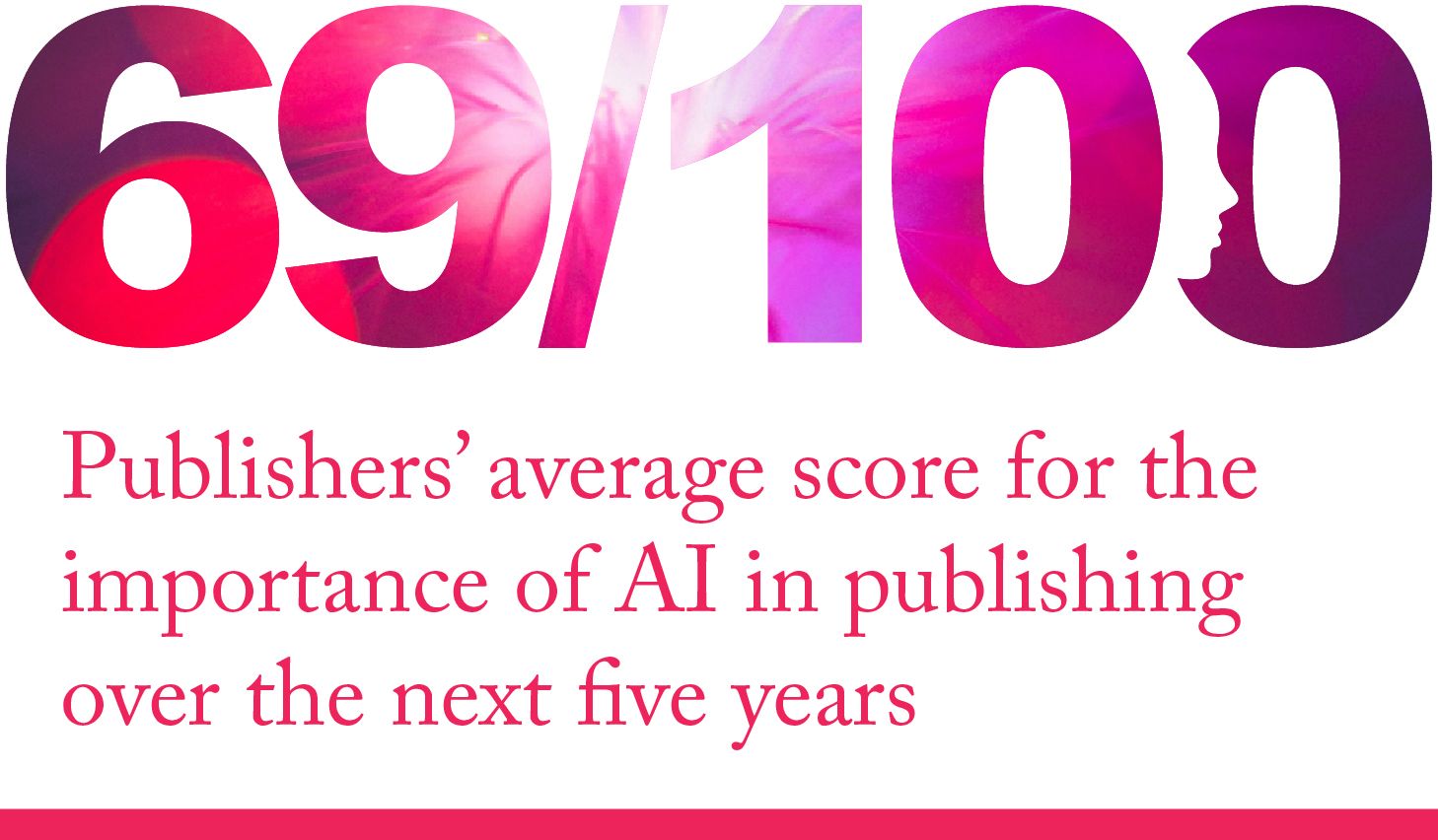
Our survey evidence suggests that AI has the potential to significantly transform publishers’ organisations. One in six publishers expect to experience significant transformation and all large publishers expect AI to have at least a small impact. It may take some time for this transformation to begin. Around half of large publishers expect transformation to begin within three years and the remainder within ten years. The scale and speed of transformation is expected to be slower by SME publishers, however: one-third do not expect AI to transform their organisation, and the majority expect that any transformation will not occur within the next five years..
AI may also have a significant impact on publishers’ competitive environment. Almost two-thirds of publishers (and 80% of large, AI-active publishers) expect to be competing with different types of organisations in the future as a result of AI. Our evidence identified AI-tech start-ups, and established tech companies turning to publishing-related AI applications, as potential future competitors.
Case study 3
AI and education publishing product development (McGraw Hill)
McGraw Hill, an education publisher, has invested significantly in AI technology. Its two AI-assisted platforms, ALEKS and SmartBook, exemplify how AI is driving significant wider benefits to educators and learners.
The ALEKS platform ( “Assessment and Learning Knowledge Spaces”) was developed in partnership by a team at the University of California and McGraw Hill, with the help of a grant from the US’ National Science Foundation. The partnership resulted in a platform that uses AI to deliver tailored content to maths, science and business school and Higher Education students. McGraw Hill acquired the ALEKS Corporation in 2013.
The mapping is carried out using Knowledge Space Theory. This draws on the idea that there are many possible states of knowledge of a human learner. AI is applied (using a series of 20 to 30 questions) to understand precisely which part of the knowledge space a student is in. This information determines which topic to teach the student next.
SmartBook, McGraw Hill’s other AI platform, was also developed externally by Danish EdTech company, ‘Area9’, and acquired by McGraw Hill in 2014. SmartBook is an adaptive reading tool. The latest iteration was launched in 2019. It augments textbook content by highlighting the most relevant text for a student and uses AI to assess students’ knowledge and guide them in reinforcing this.
McGraw Hill cites student benefits from these platforms as including increased engagement, improved study time effectiveness and higher quality learning (learning gradually rather ‘cramming’) resulting in deeper understanding and improved recall rates. Student feedback to McGraw Hill is positive. Educators benefit from saving time and so can use their classroom time more effectively. They can also access improved student progress analytics, enabling them to quickly spot trends that may take longer to feed through in larger classes, or quickly identify struggling students. The platforms are being used as a recruitment tool – for example, Brunel University promotes the SmartBook platform at Open Days.
Source: Frontier Economics stakeholder research
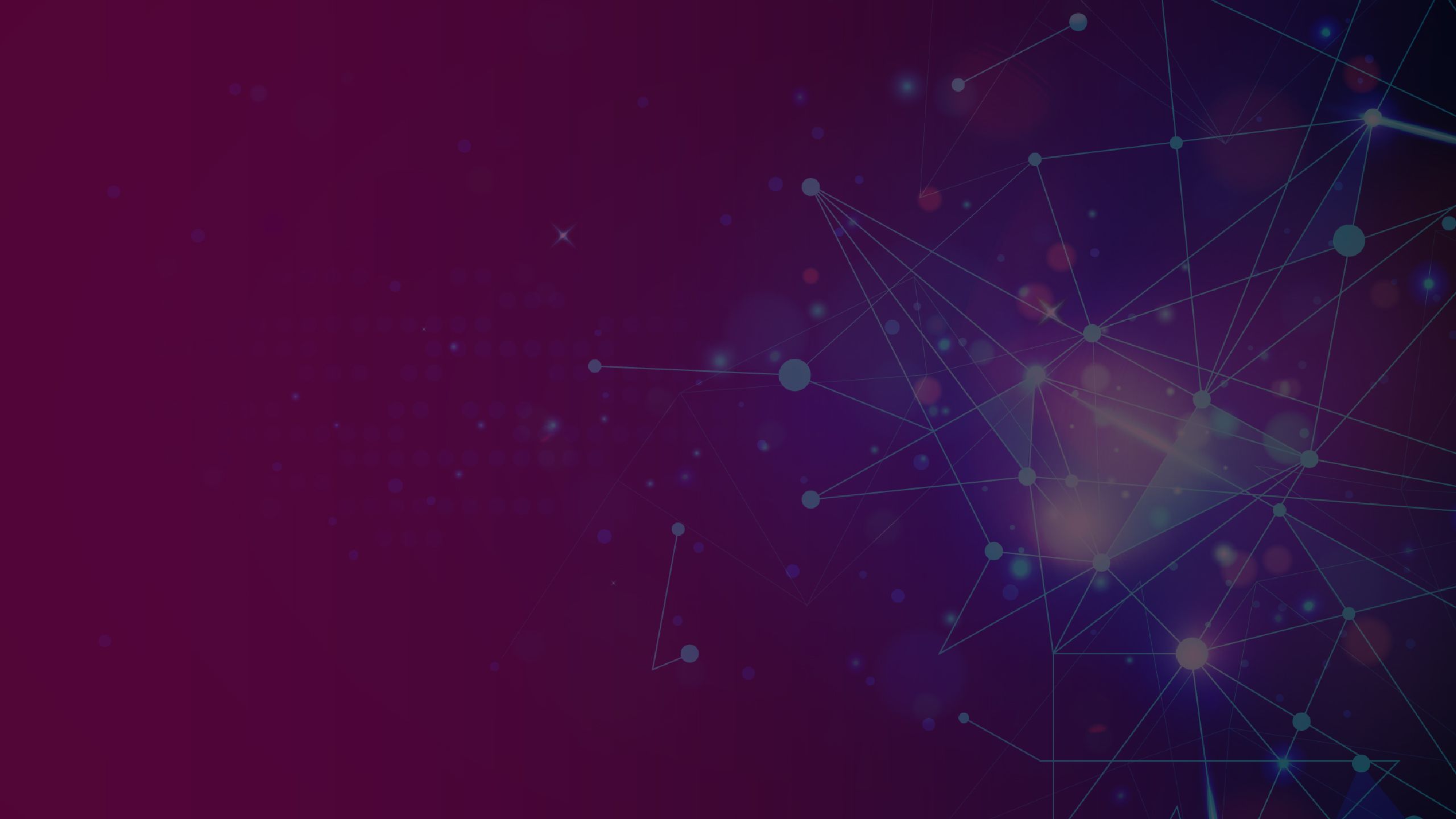
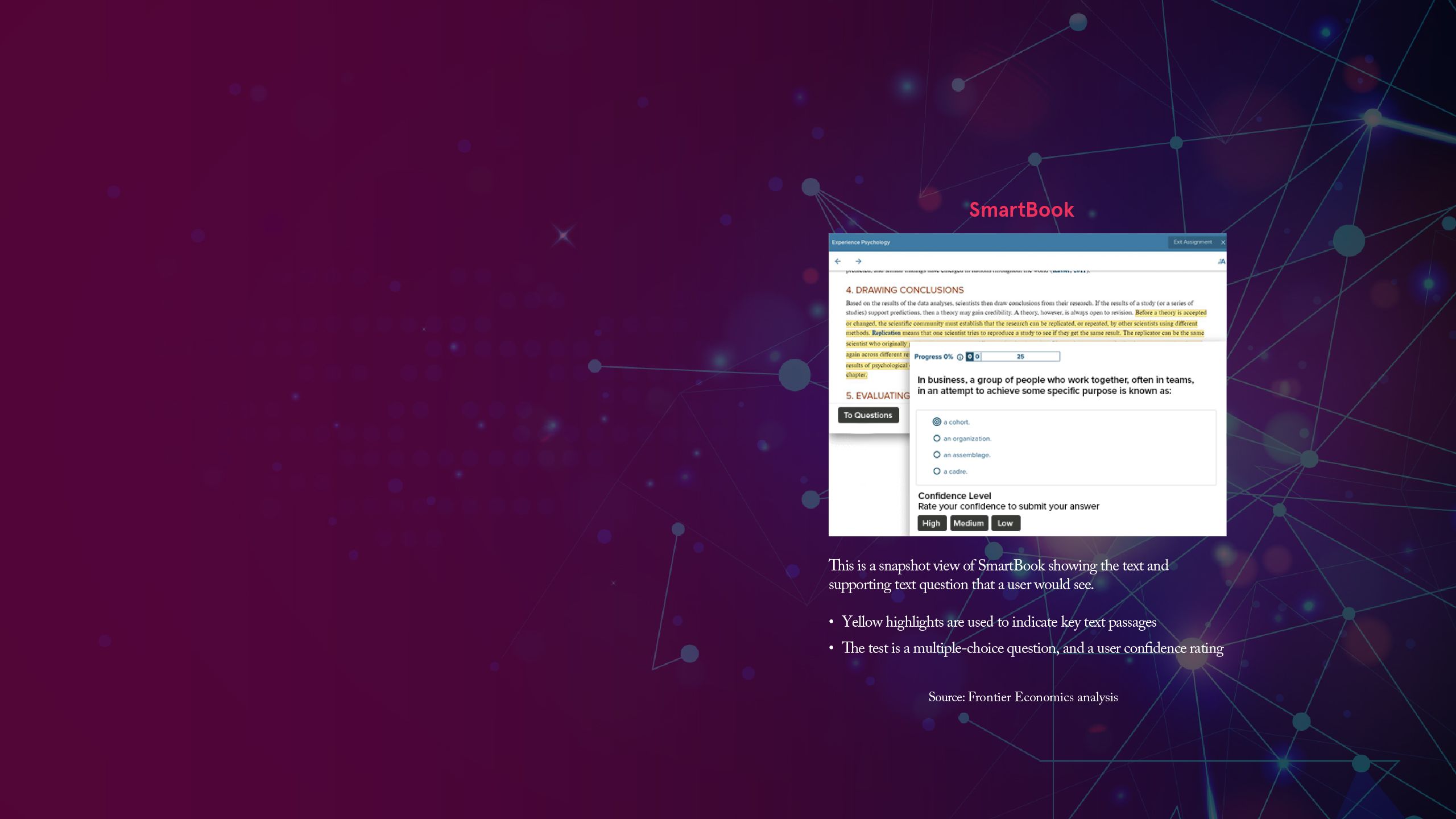
Recommendations
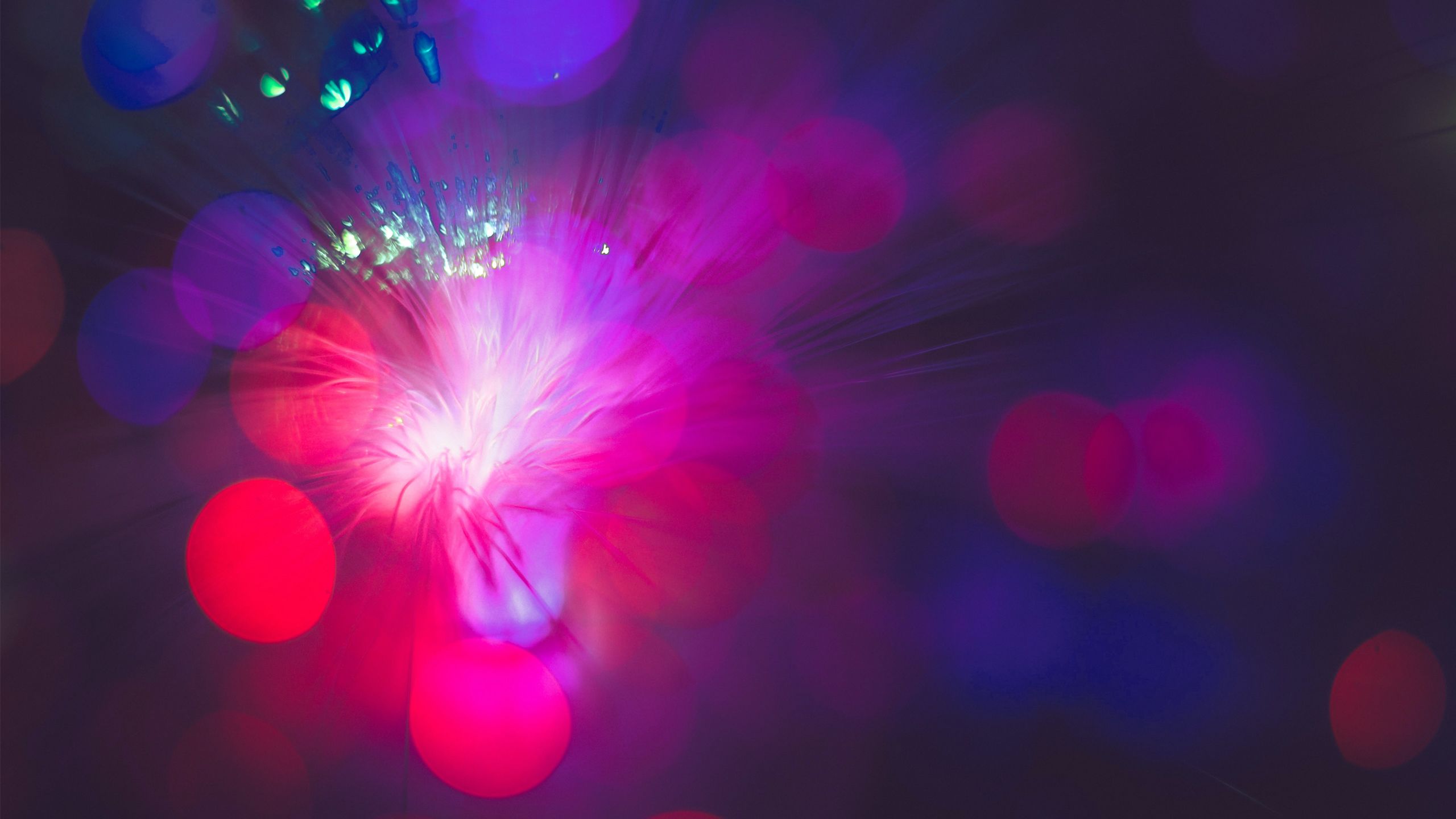
Investment in AI by publishers has the potential to generate significant benefits for the UK publishing industry and the wider economy, including productivity enhancement. AI technologies do not necessarily represent a threat to the current labour force in publishing – rather, they could be significant enablers of creativity, allowing authors, researchers and other creatives to invest less time in routine tasks and more time in generating new content. Many publishers believe these benefits are obtainable within the next two to three years, with the right levels of investment, though SMEs may be further behind.
Our research has identified three areas of concern regarding AI in publishing, which could represent key barriers. We recommend that the industry and government in the UK work together to raise awareness of the issues, promote engagement and identify policy and other solutions to address them.
- Ensuring legal certainty with regard to UK Intellectual Property law. In particular, our analysis identified the following specific concerns:
Rights issues associated with using text and data mining (TDM) to conduct research, given that publishers hold the rights to significant amounts of data that are required for TDM to be applied.
Copyright protection and legal responsibility for AI-generated works, in particular around concerns that AI technologies that summarise content or make editorial decisions could introduce bias, misrepresent information or infringe copyright in underlying content.
Patenting of AI-created content, as patents are typically awarded to incentivise human creativity yet there is no clear guidance over who is the inventor of an invention involving AI activity.
- Promoting R&D and other collaboration between publishers, AI-focused SMEs and academia: Many publishers (particularly SMEs) do not have the capacity, resources or skills to experiment with and develop AI technologies in-house, and those with some capacity still value partnership or collaborative working. Collaborative working between business and academia is at the heart of the UK Government’s Industrial Strategy.[4] The Strategy includes specific policies focused on the Creative Industries,[5] and on promoting AI-led innovation in the UK.[6] At present, though, despite our evidence on the potential of AI in a key part of the creative economy, publishing’s voice appears to be relatively unrepresented in this critical policy debate. We recommend that the publishing sector engages strongly with the developing Industrial Strategy, ensuring that the industry is fully represented in future policy discussions which will enable publishing’s needs to be better addressed with tailored support. Government should also actively engage directly with the sector – for example, encouraging participation in bidding for collaborative funding through Challenge Funds working with academics and tech-focused SMEs to drive and enable future AI-led innovation.
- Helping publishing SMEs access AI investment finance and skills: Our industry survey suggests that SME publishers (particularly micro-enterprise) recognise the potential benefits of AI investment but lack the resources they need to invest. The costs of researching, acquiring and implementing AI solutions and AI skills were identified as significant investment barriers by SME publishers that responded. We recommend further engagement between the Publishers Association and its SME members, for example through a taskforce focused on addressing SME AI investment issues. Evidence from this should inform future engagement with government exploring how to improve SME’s ability to invest in and benefit from cutting edge technologies such as AI.
[5]
https://www.thecreativeindustries.co.uk/media/462717/creative-industries-sector-deal-print.pdf
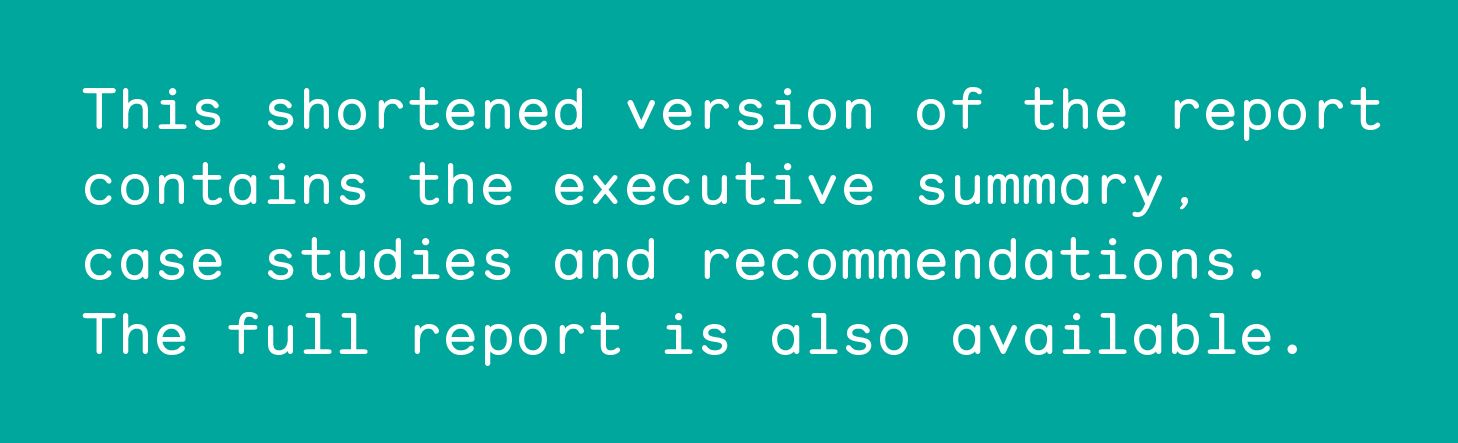
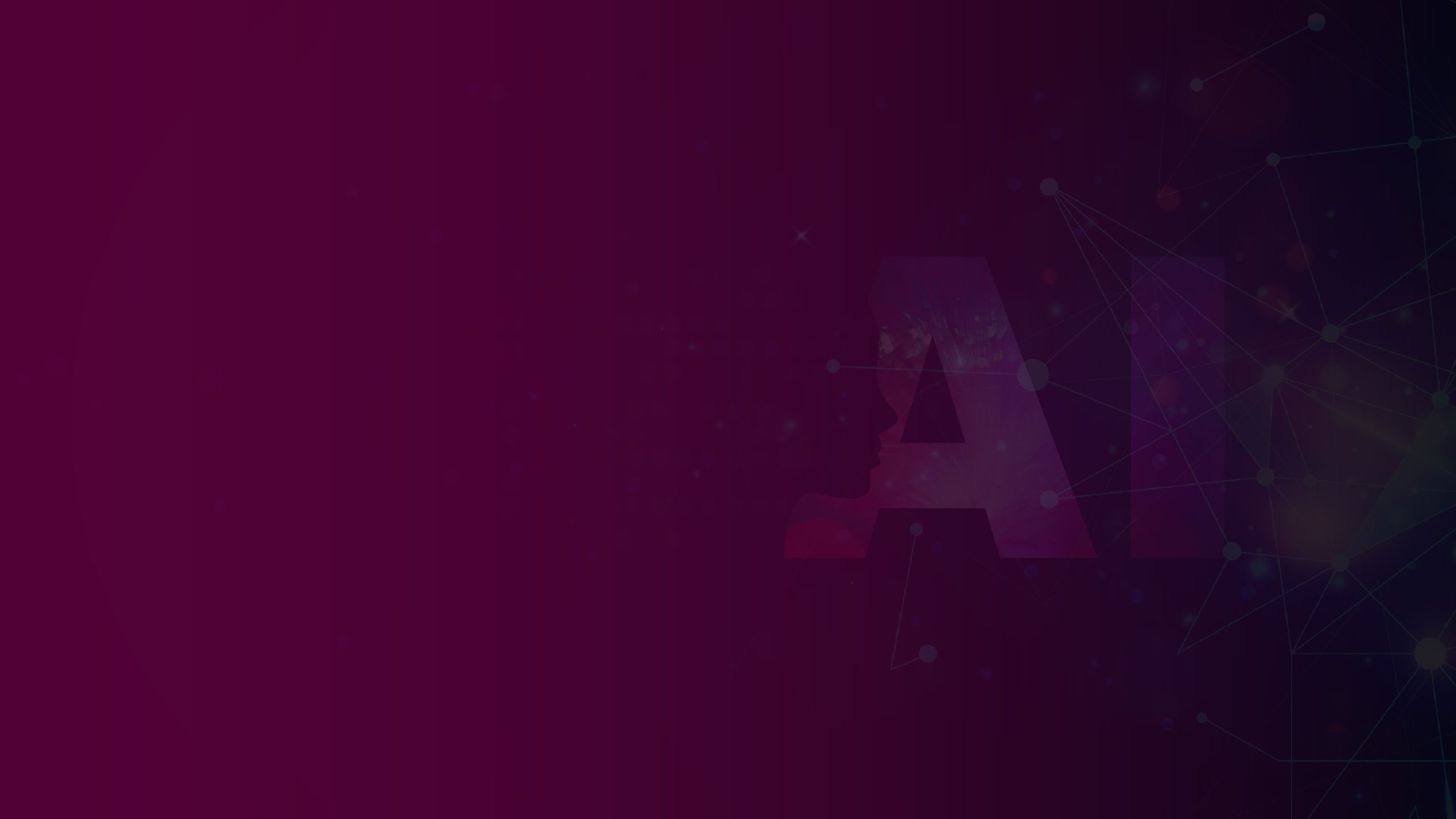
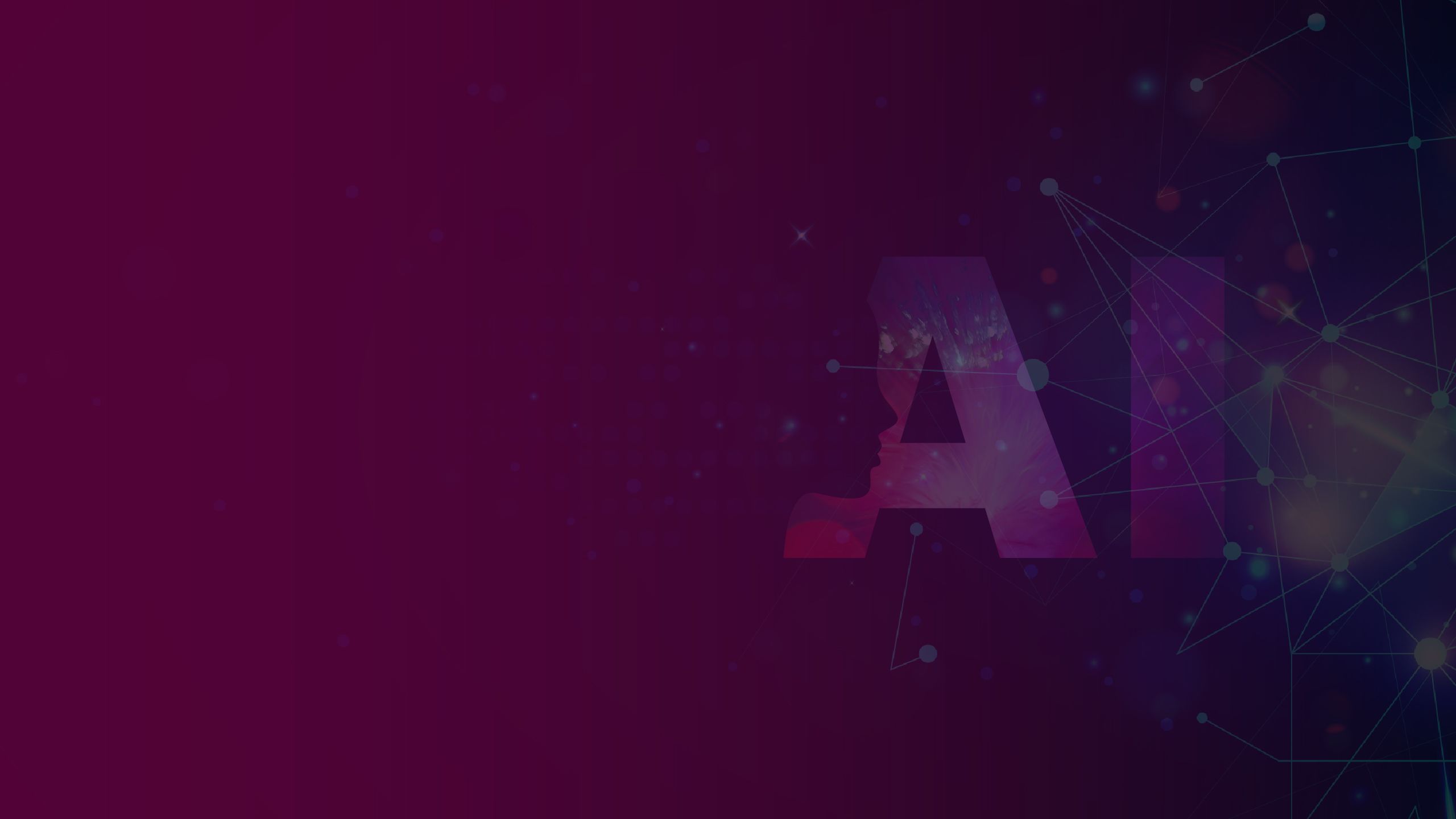
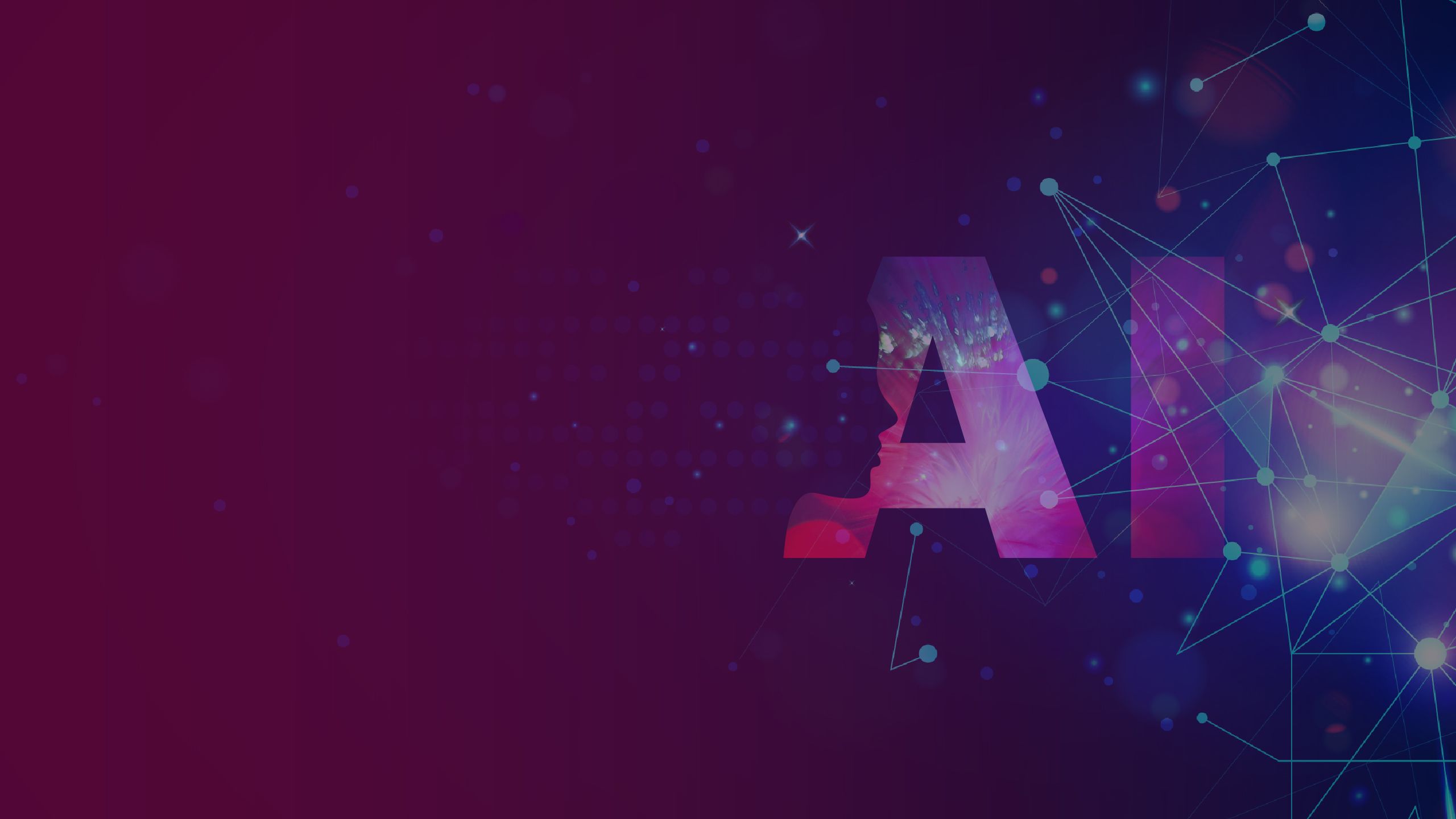
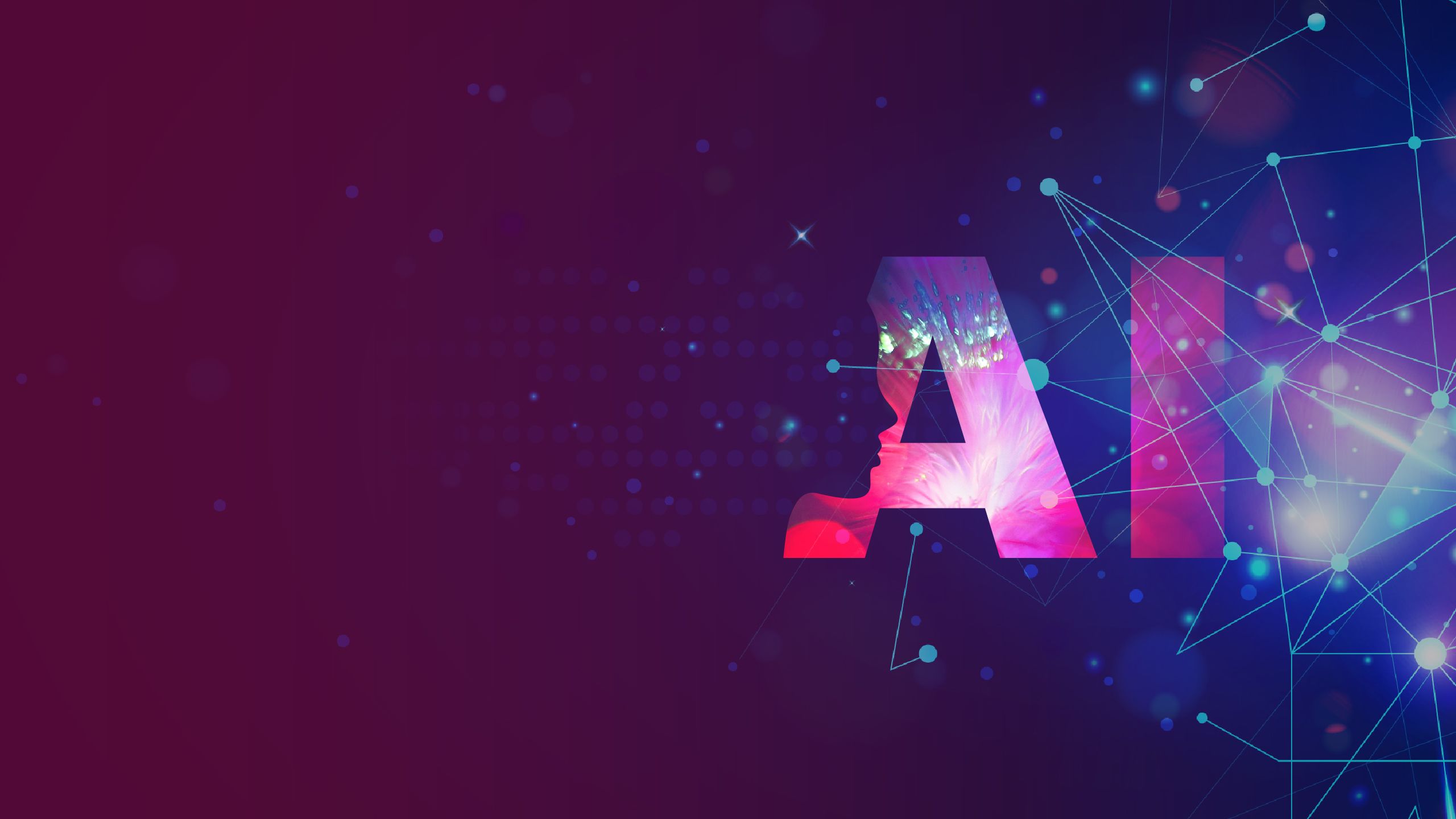